Can you imagine a world without the kind of voice assistant technology provided by Amazon Alexa, Google Assistant, Siri on the iPhone or Cortana for Windows? Probably not, as we tend to take such technological leaps forward pretty much for granted. But behind the scenes there’s a whole new world of machine-learning that drives their collective ability to seemingly answer any question put to them. It’s not so much knowing the answer that’s the technological miracle – because, well, the internet – but rather that these virtual assistants are able to understand the question in the first place.
We need to be thoughtful about how AI technology can be used to enable responsible innovation
Machine-learning is, in the broadest possible terms, what you might expect in that computer algorithms can be trained to understand how to correctly respond to an input by way of a human telling it what that response should be. Over time the collective inputs and outputs enable the computer to learn, albeit within a relatively narrow and defined speciality, all thanks to the skilled operators that handle this education.
Which is where one of the biggest conundrums surrounding AI pops up: the paradox that it will solve the skills shortage across multiple industry sectors yet requires skilled operators (who are in very short supply themselves) in order to achieve it. But what if the machines could teach themselves?
Is unsupervised machine-learning really possible?
When talking about the machines teaching themselves, this is what’s known as unsupervised machine-learning. The simple definition of which is that the machine, or rather the algorithm running it, can be trained without the classification or labelling of data. In other words, the algorithm takes the input and determines the correct response itself, without the need for the output confirmation part of the learning equation.
But have we really reached that far in the development of thinking machines? Labhesh Patel, chief scientist of Jumio, answers with a qualified yes. Mr Patel, whose company uses machine-learning to deliver identity verification and authentication solutions, says that the best machine-learning models start with supervised training and once the AI system is regularly outputting correct results then unsupervised models become a viable proposition.
“Think about sites like Amazon or Netflix that offer recommendations,” Mr Patel explains, “once the machine-learning models have been created, they will learn based on user behaviour. If a recommendation keeps getting clicks, those clicks will feed the intelligence of the algorithms and that recommendation will become more prominent.”
Another example is language modelling, according to Dr Tom Ash, a machine-learning engineer with speech recognition company Speechmatics. Language modelling involves “learning about grammar and sentence structure from large bodies of text without any labelling of those words into different classes,” he says. In other words, algorithms can be applied to identify patterns in random data without the need for human input.
“This perspective is hugely interesting as human beings live in a world full of patterns,” says Antonio Espingardeiro, Institute of Electrical and Electronics Engineers member and an expert in the field of robotics and automation. “Humans tend to observe and generalise,” Mr Espingardeiro says. “These algorithms apply formulas and present possible results without generalisation.”
Will AI only ever be as good as its human teacher?
Such an ability comes to the fore in areas where there is either a lack of ‘pre-labelled’ data or a requirement to minimise the bias that ‘expert knowledge’ inevitably brings with it. “Without target information to train on, the learning algorithm must learn from general patterns inherent within the data,” says Chahmn An, principal software engineer for machine-learning at next-generation threat intelligence provider Webroot, who continues: “such as the shape of edges within images or syntax patterns within natural language.”
Not everyone agrees, however, and some insist that it’s a myth to suggest an AI can teach itself anything outside of the context of its learning environment, which means it is only as good as the combined forces of the human teacher and the data it is fed. And there is certainly an argument to be made that, currently at least, human oversight is required to ensure that machine-learning-driven decisions are transparent and trustworthy.
“An insurance AI let loose on unstructured driver data might decide blonde people are a higher risk of having accidents because they happened to be over-represented in the sample of risky drivers,” argues Ben Taylor, chief executive at Rainbird, which helps deploy AI across multinational corporates. Mr Taylor points out that the insurance industry is not alone in having poor data hygiene, which could lead to unsupervised AIs making critical errors.
Could self-teaching machines help end the skills shortage forever?
But what of that skills shortage paradox mentioned earlier; will unsupervised machine-learning help to break out of this seemingly unending contrary loop? Mastercard has been pioneering the use of AI that tests the algorithms used to detect fraud and anomalies in payments, and has had some success using AI that monitors other AI models through unsupervised learning techniques.
Ajay Bhalla, president for cyber and intelligence solutions at Mastercard, is certain that machine-learning will plug the skills gaps across a range of industries, at both the skilled and unskilled ends of the spectrum. “By supporting the performance of routine tasks, AI tackles ‘thought labour’ for high-value roles, freeing up experts to do the critical thinking machines simply can’t do,” he says.
But that doesn’t mean that unsupervised machine-learning is automatically a win-win. “We need to be thoughtful about how AI technology can be used to enable responsible innovation,” Mr Bhalla says. “This will inevitably require greater collaboration and transparency as solutions advance, as well as attention to how data is handled and how we address issues such as unintended bias.”
And while nobody is suggesting a Skynet scenario here – the fictional AI network that features as the main antagonist in the Terminator film franchise, for those who are not familiar – issues surrounding misuse of data and the potential for bias that could have negative consequences for customers and companies alike, cannot be ignored.
“The release of the EU’s ethical guidelines for trustworthy AI is a step in the right direction,” concludes Peter van der Putten, assistant professor of machine-learning and creative research at Leiden University and global director of AI with customer relationship software outfit Pegasystems. “But it will be up to the individual providers to comply and iron out any ethical issues with the AI they are using before it is fully implemented.
Is unsupervised machine-learning really possible?
Will AI only ever be as good as its human teacher?
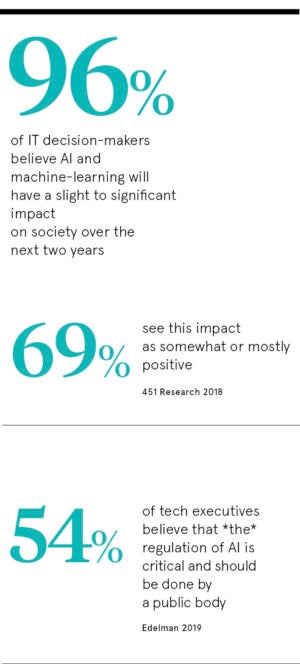