Data is pouring into companies in torrents, bearing unstructured information about markets, customers, resources and trends. Businesses know that it’s something to be harnessed rather than feared, and are looking to artificial intelligence and machine-learning (AI/ML) to scry insights and value.
AI/ML is far from a fit-and-forget technology. For any business to embark on unstructured data-driven AI/ML, a lot of questions need answering. Starting with what is AI/ML in a business context?
The ability to link unstructured and structured data is where the value lies; you get new insights and reveal unknowns
“The best definition of AI I know is making machines do tasks that require human intelligence,” says Fern Halper, vice president of research at data research and education company TWDI. “Companies have been using predictive analytics to do that in some ways for decades, but the uptake hasn’t increased. Now we’re in the early mainstream of AI/ML, that’s going to change.”
Pairing structured and unstructured data to reveal deeper insights
The most important thing, she says, is knowing what you want to do. Many of the companies TWDI survey are concerned with understanding customer sentiment, so are using natural-language analysis on unstructured sources such as email, customer reports and social media.
“Some 25 per cent are extracting sentiment and combining that with structured data from billing and service history to build a predictive model of who’ll buy what,” says Ms Halper. “The results are better than you can get from structured data alone.
That sweet spot of identifying where AI/ML can extract meaning from unstructured data that then reinforces structured information is a productive one, says Nick Lynch, consultant at the Pistoia Alliance, a pharmaceutical industry not-for-profit organisation that identifies and develops cross-company techniques and tools.
“Unstructured data is a field in transition,” he says. “The health industry has lots of it, from early science to doctor’s notes, images, histories, formulae, genetics. It’s very valuable, but deriving interesting things from it is hard. Industry is making big improvements in unstructured data and the ability to link it to structured data is where the value lies; you get new insights and reveal unknowns.”
Knowing whether you have the right data, what the right approach is and whether the results are working are all early and important stages on the path to AI/ML. That needs good people or good partners, says Ms Halper. “Talent is the number-one challenge,” she says. “Then comes getting good quality data and integrating it. Here, all the vendors are trying to make it easier for anyone, not just developers, to use AI/ML and put it in apps. Regardless of how easy this is, explainability is very important. You need to explain how your model works for compliance and to foster confidence within your company. Is your model defendable? The market is moving this way.”
How AI/ML can create value by replacing humans in mundane tasks
Trust is important, both for buy-in within a company and when providing high-value, high-risk results such as diagnoses from medical images. The same is true where AI/ML is used to automate the most mundane, everyday tasks, says Marcos Jimenez, chief data scientist and co-founder of X.AI, which has developed an AI called Amy that arranges business meetings.
Amy is an example of another growing class of AI/ML, the agent or bot that replaces humans in an interactive task. “Amy is a task-oriented dialogue engine. It understands commands like ‘set up a meeting with Jim and Sally’ and arranges it by email,” says Mr Jimenez. It’s a precise example of automating a task that uses human intelligence and one that was created with a great deal of human input building another vital aspect of AI/ML, domain knowledge.
“We looked at thousands of situations, extracting elements for natural-language processing. Initially, humans in the loop called AI trainers labelled stuff to kick-start the process. We used ML to create datasets that characterise meetings from hundreds of thousands of hand-labelled emails. It was super-expensive to build,” he says.
The AI then knew what a meeting was and how humans talked about it. After this, adjusting a meeting to what people wanted was a much easier task, as was creating a natural-language output system that could use email to get or send information.
How machines can tackle the ultimate unstructured data source: text
Text is the ultimate unstructured data, says Mr Jimenez; you never know what you’re going to get and it’s never the same as what you’ve seen before.
“You have humans in the loop at first and, as your model gets better, you can move them out,” he says. “Eventually, the customer becomes the human in the loop because human language always has ambiguity and incomplete cases. Amy is trained to ask for clarification when this happens, but that’s exactly what humans do. We measure Amy’s performance against that of humans doing the same task and it’s just as good, so we have a product.”
Your company doesn’t have to be so AI/ML focused, says Ms Halper, but as awareness grows there can be unexpected benefits. “I see a virtuous circle, a success cycle,” she says. “You start to see how your infrastructure can work with all sorts of data, and how all techniques like data warehouses, data lakes, cloud and virtualisation can support AI/ ML The company becomes more data aware, more confident with analytics and new applications become apparent.”
Expect some pain at the beginning, she advises, but pick early tasks that can show obvious success and grow from there. “Data is alive, organic; it’s not structured. Evolving the tools and understanding to use it won’t be optional for long,” Ms Halper concludes.
Pairing structured and unstructured data to reveal deeper insights
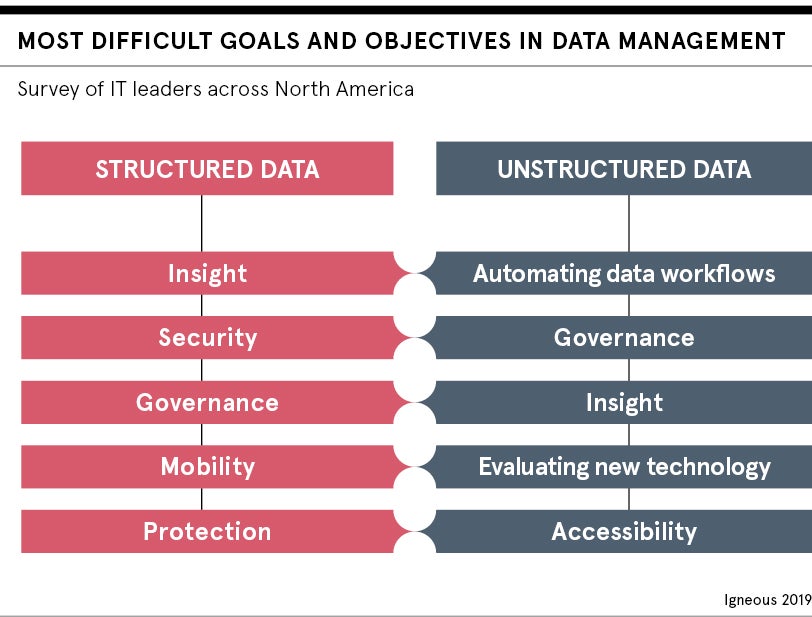