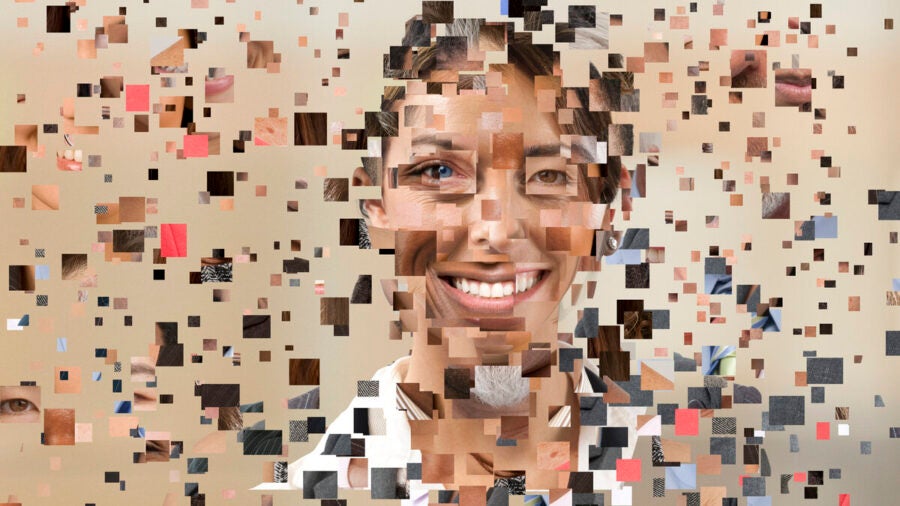
Any AI system, however sophisticated, is only as good as the data on which it is being trained. Any bias in its outputs will result from distortions in the material that humans have gathered and fed into the algorithm. While such biases are unintentional, the AI sector has a predominantly white male workforce creating products that will inevitably reflect that demographic group’s particular prejudices.
Facial recognition systems, for instance, could be inadvertently trained to recognise white people more easily than Black people because physical data on the former tends to be used more often in the training process. The results can put demographic groups that have traditionally faced discrimination at even more of a disadvantage, heightening barriers to diversity, equity and inclusivity in areas ranging from recruitment to healthcare provision.
An AI arms race
The good news is that the problem has been widely acknowledged in business, academia and government, and efforts are being made to make AI more open, accessible and balanced. There is also a new ethical focus in the tech industry, with giants including Google and Microsoft establishing principles for system development and deployment that often feature commitments to improving inclusivity.
Nonetheless, some observers argue that very little coordinated progress has been achieved on establishing AI ethical norms, particularly in relation to diversity.
Justin Geldof, technology director at the Newton Europe consultancy, is one of them. He argues that “governments have abdicated responsibility for this to the tech industry and its consumers. There is no formal watchdog and no general agreement on norms in AI ethics. With no one stepping in to lead on AI and its ethics, this has in essence become an arms race.”
The new frontier in AI
Recent breakthroughs in the field of generative AI have also done little to address concerns about discrimination. In fact, there’s a risk that the potential harms of generative systems have been forgotten in all the media hype surrounding the power of OpenAI’s ChatGPT chatbot and its ilk, argues Will Williams, vice-president of machine learning at Speechmatics.
With no one stepping in to lead on AI and its ethics, this has in essence become an arms race
Meta took Galactica, its large language model, offline in November only three days after launch, for instance, amid fears about its inaccuracy and potentially dangerous impact on society.
Williams says: “The truth is that the inherent bias in models such as ChatGPT, Google’s Bard and Anthropic’s Claude means that they cannot be deployed in any business where accuracy and trust matter. In reality, the commercial applications for these new technologies are few and far between.”
In simple terms, generative AI models average the opinion of the whole internet and then fine-tune that via a process known as reinforcement learning from human preferences. They then present that view as the truth in an overly confident way.
“This might feel like ‘safe AI’ if you are one of the humans fine-tuning and editing that model,” Williams adds. “But, if your voice isn’t represented in the editing room, you will start to notice how your opinion varies dramatically from those of ChatGPT, Bard and Claude. Your truth might be some distance from the truth they present.”
Removing the bias
The race to produce a winner in the generative stakes has given new urgency to addressing bias and highlighting the importance of responsible AI.
Emer Dolan is president of enterprise internationalisation at RWS Group, a provider of technology-enabled language services. She says that, while the detection and removal of bias is “not a perfect science, many companies are tackling this challenge using an iterative process of sourcing targeted data to address specific biases over time. As an industry, it’s our duty to educate people about how their data is being used to train generative AI. The responsibility lies not only with the firms that build the models but also with those that supply the data on which they’re trained.”
Wider technical, analytical and academic communities are applying several methods to reduce or remove bias. These include supervised learning; synthetic data sets containing computer-generated material rather than real-world data; and peer reviews, in which teams with no prior knowledge of the data analyse the code and the results.
“Machine learning models that banks are using in areas such as fraud detection have too often been based on relatively small samples,” says Ian Liddicoat, CTO and head of data science at Adludio, an AI-powered advertising platform. “To achieve more accurate results, synthetic data can be used to mimic and augment the original data sets. This data also seeks to even out the distributions for factors such as gender to ensure that they reflect society more accurately.”
Data engineers are also using more advanced random-sampling methods that create data sets for modelling, where each record has an equal chance of selection. Supervised learning methods, meanwhile, can be applied to neural networks, so that real-world distributions for factors such as race or gender are enforced on the model.
“Another effective solution”, Liddicoat adds, “is to use sub-teams to conduct detailed peer-to-peer reviews of the input data, the machine-learning method, the training results and the operational outcomes.”
Beyond the technical challenges of removing bias from the systems and the data they’re based on lies the bigger matter of improving diversity in the AI sector. The only truly effective way to achieve this is for employers in this field to recruit from a wider talent pool. The ongoing lack of diversity in academia, particularly in STEM subjects, is troublesome for the future of AI. Until that fundamental problem is solved, AI may always pose a threat to diversity, equity and inclusivity.
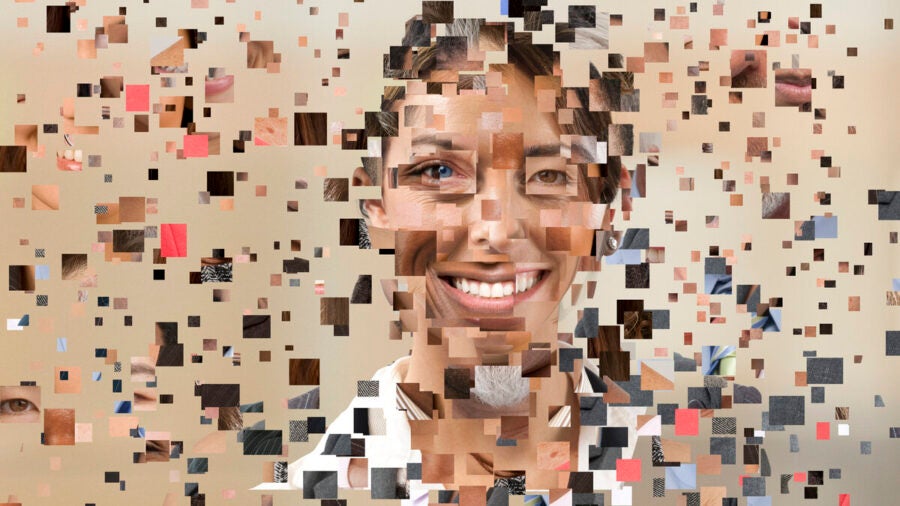
Any AI system, however sophisticated, is only as good as the data on which it is being trained. Any bias in its outputs will result from distortions in the material that humans have gathered and fed into the algorithm. While such biases are unintentional, the AI sector has a predominantly white male workforce creating products that will inevitably reflect that demographic group’s particular prejudices.
Facial recognition systems, for instance, could be inadvertently trained to recognise white people more easily than Black people because physical data on the former tends to be used more often in the training process. The results can put demographic groups that have traditionally faced discrimination at even more of a disadvantage, heightening barriers to diversity, equity and inclusivity in areas ranging from recruitment to healthcare provision.