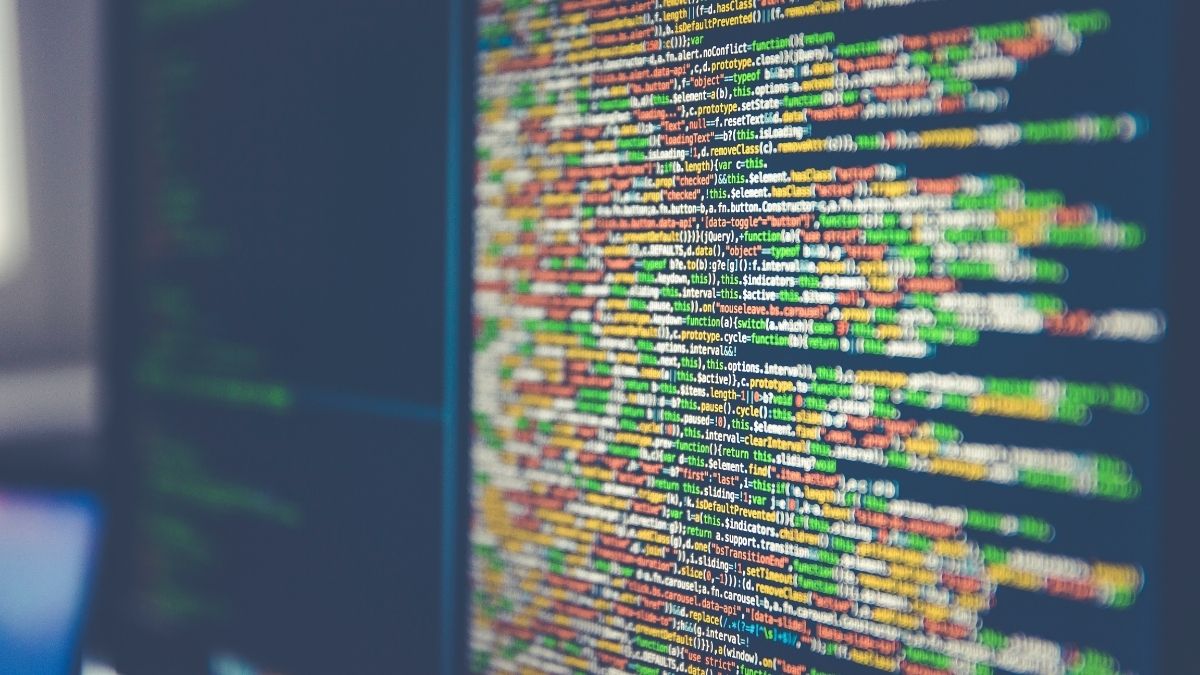
Artificial intelligence is everywhere. If a business isn’t using AI, then it’s either claiming to use it or claiming that it’s about to start any day now. Whatever problem your company is having, it seems that a solution powered by decision intelligence, machine learning or some other form of AI is available. Yet, beneath the marketing hype, the truth is that many businesses can indeed benefit from this tech – if they take the time to learn what it can (and can’t) do for them and understand the potential pitfalls.
In essence, AI enables its users to do useful things with a large pool of data – for instance, fish out insights without tying up the time of data scientists. Data is therefore fundamental to AI. There is a direct relationship between the quality (and quantity) of what’s fed into a machine-learning application and the accuracy of its output.
Data governance has traditionally been viewed in terms of complying with regulations that stipulate how data must be collected, stored and processed. But AI has introduced new challenges and risks to be managed. It’s not enough to obtain a vast amount of data; you also need to consider its characteristics. Where is it coming from? What does it actually represent? Is there anything that you need to account for before feeding this material into your algorithm? Will it train the algorithm in the right things?
“We can use AI to identify unusual patterns of behaviour in a business… or we can see a business changing how it earns money from contracts in real time,” says Franki Hackett, head of audit and ethics at data analytics firm Engine B. “To do this, you obviously need a clear idea of what is relevant, along with high-quality governance processes over your AI. Otherwise, you find either that there are far too many ‘risky’ items to consider or that the AI points you in the wrong direction.”
More input required
One way of approaching data governance is to use a tool known as an observability pipeline. This ensures that every process is visible, collecting data that is then unified and cleaned up to create a more consumable final data set.
An example would be the conversion of raw website logs to an analytics platform. The original data and its point of consumption are ‘buffered’ by the pipeline: the raw data enters it and is processed before being sent out to where it needs to be consumed. The method of consumption can easily be altered because the underlying data is unaffected – that is, you can change how the data is presented without changing the collection process.
AI can both benefit from this process and become part of it. The pipeline itself may feed an algorithm, but machine learning can be used to detect anomalous data (based on past trends) before it gets too far. This can save people the time and effort they would otherwise need to spend on checking and cleaning data and, once it’s been processed, investigating any irregularities. But it can also ensure that business-critical algorithms aren’t being fed data that will lead them to draw the wrong conclusions and, potentially, nullify any benefits gained from introducing AI to the process in the first place.
Ensuring observability has plenty of benefits for data flows that don’t involve AI, but the sheer volume of material involved and the complexity of machine-learning processes mean that it’s vital to know what’s happening to the data being processed. Checking that the number of visitors in your web analytics matches what your logs tell you is trivial compared with understanding the output of a complex algorithm that’s being trained and tuned over time.
If a data set and model is considered too accurate in its score, it could lead to over-representation, making things go horribly wrong
This is because a system that might have started by providing the insights you were seeking could be drifting ever further away from generating anything useful. The better your view of what’s happening to the data, the easier it is for you to prevent this outcome.
The risks in this respect can be more serious than, say, the potential overstatement of a set of projected sales figures. Dr Leslie Kanthan, co-founder and CEO of AI firm TurinTech, offers an example of where the stakes are much higher: “If AI is applied to a hospital’s magnetic resonance imaging scans and it misdiagnoses a serious disease such as pulmonary fibrosis as bronchitis, causing the patient to take incorrect medication and experience adverse side effects, who is to be held legally accountable?”
He continues: “Similarly, if a data set and model is considered too accurate in its score, it could lead to over-representation, making things go horribly wrong. For example, an AI model that is used to predict future criminal behaviour could overfit data and incorrectly come up with a bias against ethnic minorities.”
Data governance is key to ensuring that AI produces useful results. It incorporates an understanding of not only ethical and legal issues but the implications these have for what material must be collected and its potential limitations.
The organisations that will benefit the most from AI will be those that take the time to build a framework that ensures they’re targeting the right data; collecting enough of it; checking and cleaning it to ensure that it’s of a high standard; and then using it in an appropriate, ethical way.
With the right data governance in place, these enterprises can maximise the benefits and minimise the risks of using AI to provide insights that will streamline their processes, inform their decision-making and create powerful new products and services. There is a lot more than hype behind what AI can do for your business – as long as you lay the right foundations for it.