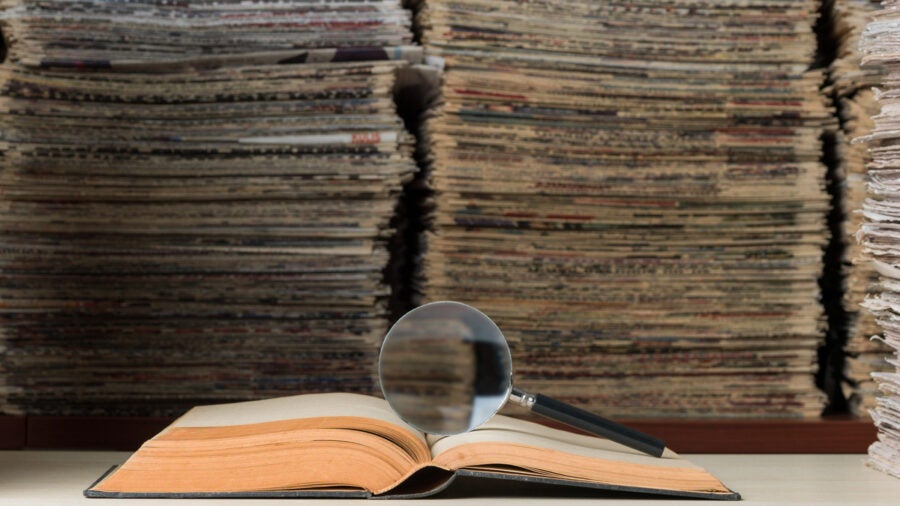
Large language models (LLMs) such as ChatGPT are generating lots of hype in business analytics. Ben Hookway, Relative Insight’s CEO, talks about what LLMs can do now, what’s missing and how they could be improved.
Q How do you view the market for large language models and generative AI in business analytics?
A The hype cycle starts with inflated expectations, followed by disappointment and then equilibrium when people figure out what works and what doesn’t. People are at different points in this cycle. They are also at different sophistication levels, depending on their experience with AI.
But we’re talking to lots of senior leaders in consumer insight, analytics, customer experience and employee engagement about AI technologies.
Q What are the limitations of LLMs and how could they be more useful?
A Without measurement, evidence and audit, models like ChatGPT add to the noise and volume of unstructured information in business analytics.
At Relative Insight, we have proprietary AI applications in consumer insights, customer experience, and employee engagement. We analyse content such as call transcripts, live chat and surveys with open-ended questions. Our AI can give you a sophisticated analysis of the answers, with much more information about why, for example, your net promoter score is going up or down, why people buy from you and why employee satisfaction scores have changed.
Those explanations might once have been anecdotal or shown as a generic word cloud. Now, we have a more efficient and robust way of analysing the information based on comparison.
Our AI technology grew out of a law-enforcement project to identify child abusers who were masquerading online as young teenagers. The technology identified a 4% difference in their language compared to verified 13-year-olds. That difference was what we needed to catch the offenders when other methods could not.
We’ve translated that capability to a business context, comparing and analysing text between customer and employee segments.
Q How does it work?
A You might know a lot about your customers based on income, age, geography and so on. Say you want to target younger people. Instead of using a bland word cloud, it’s much more useful to use AI to compare how people in their 20s and those in older age groups talk about your service.
Our proprietary model can identify what that cohort says about your product that is significantly different from other age groups. Or what people who gave it a three-star rating are saying that’s different from those who gave it four stars. Or the changes since you implemented your customer experience initiative to measure the impact.
That analysis is 10 times more valuable than the results obtained using the previous methods. And we express those differences as a metric. For example, we can tell you that this month people who called your customer service line talked about pricing 1.4 times more than they did in the previous six months – you can then delve into what they are talking about and discover it’s because of frustration about a specific price rise for one of your products. That helps you quickly identify patterns that can support your decisions. Now you know what’s happening and precisely why, and you can align your business strategy around the metrics. That makes your analysis stick with senior stakeholders. It’s powerful.
ChatGPT’s summaries are useful. But you can’t make confident decisions and align business activities based on a paragraph of unstructured text.
Q Could you give a more detailed practical example?
A A mobile phone retailer was under pressure to connect better with younger customers in their marketing. Relative Insight compared how older and younger groups talked about their phone and how they used it day to day, which highlighted statistically significant differences. We showed that younger people used the word ‘camera’ and the phrase ‘camera quality’ 1.2 times more than the older groups. They were also the only age group to say that they ‘shot images’ on their phones and didn’t use the phrase ‘take pictures’. The retailer then went on to highlight the phone’s camera features in its advertising and communications. Using generative AI to summarise the verbatim material, then add metrics and combine it with narrative is lighting people up as it enables them to affect outcomes much more quickly.
Q How does the technology apply in consumer insights versus customer experience and employee experience?
A In essence, the process is the same. Regardless of the data we’re analysing, we’re still helping to derive value from text data to inform people’s decisions. A recent example of employee experience is a customer who had worked very hard to improve employee wellbeing through HR-driven initiatives and we used their employee pulse survey analysis to track the themes associated with wellbeing and show improvement. When that information was benchmarked against company data on resignations, it showed a real financial benefit to the business.
Q Some large companies are pausing their adoption of LLMs for analytics. Why?
A As well as the lack of metrics, evidence and audit are missing from some LLMs such as ChatGPT.
If your analytics support high-consequence or non-reversible business decisions, executives will interrogate your conclusions and the evidence behind them rigorously – even more so if you’re using AI.
Some LLMs are black boxes. You cannot interrogate them about how they arrived at a conclusion. For some things, that doesn’t matter. But if you’re spending millions on a project, you need the evidence.
Our approach is evidenced and auditable, enabling you to understand why the model arrived at a conclusion.
Regulation always struggles to catch up with technology, particularly AI. But in a heavily regulated industry such as healthcare or finance, business decisions must be audited so you can show how you arrived at each decision that affects your customers.
To find out how to unlock data that can be used to inform strategy, please contact Relative Insight.
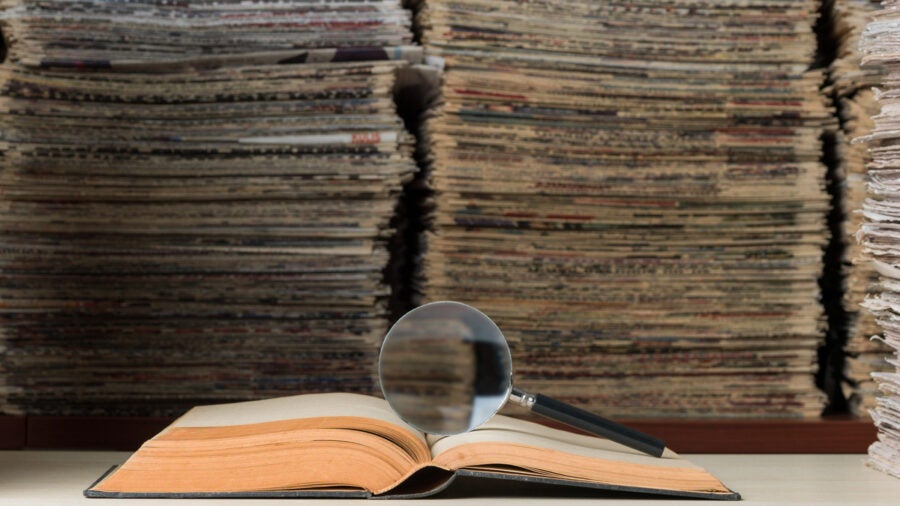
Large language models (LLMs) such as ChatGPT are generating lots of hype in business analytics. Ben Hookway, Relative Insight’s CEO, talks about what LLMs can do now, what’s missing and how they could be improved.
Q How do you view the market for large language models and generative AI in business analytics?
A The hype cycle starts with inflated expectations, followed by disappointment and then equilibrium when people figure out what works and what doesn’t. People are at different points in this cycle. They are also at different sophistication levels, depending on their experience with AI.