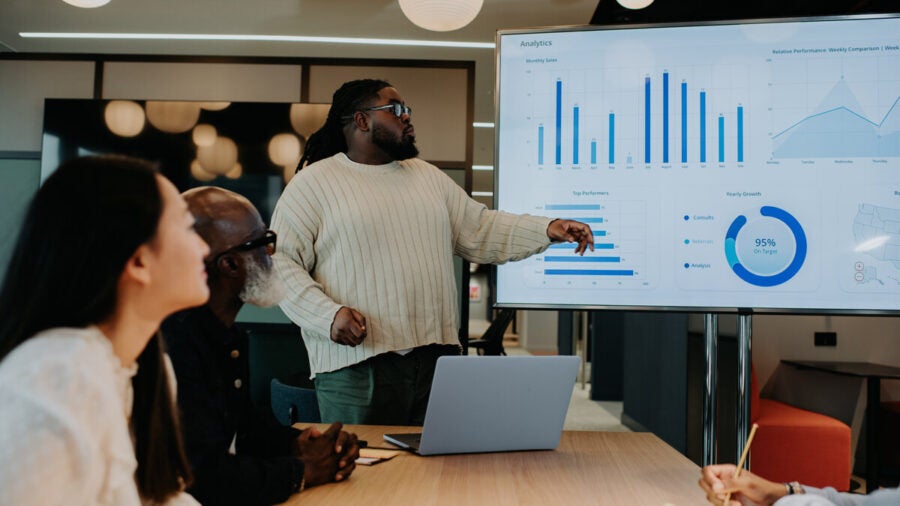
The business landscape is strewn with the corpses of corporations that failed to notice what competitors were up to, with every decade throwing up a new, high-profile victim of the head-in-the-sand approach to market share.
Think of Blockbuster’s dismissive attitude towards Netflix in the noughties and then even Netflix’s lack of foresight in seeing the streaming wars on the horizon.
This is why organisations need robust competitive intelligence (CI) strategies: to ensure that they are not blind-sided by competitors or disruptive business models.
But gathering data as part of CI can be daunting because of the amount of information available. Industry experts’ blogs or presentations, financial reports, news media items, public data sources and more are all there for harvesting.
New AI and machine learning techniques can streamline and accelerate this lengthy task. SaaS platforms use AI to track and collect historical and real-time data insights, which allows businesses to use information culled from competitors’ digital footprints.
Using data in decision-making
“There is exponentially more information online in the digital footprint of every business relative to what there was a decade ago,” explains Jonah Lopin, founder and CEO of Crayon, a competitive intelligence software platform.
“If you have the tools to aggregate and analyse this information you can understand a competitor’s product life cycle, pricing, team dynamics, latest investments and even areas where they might be struggling. Those insights can help a company to anticipate that competitor’s moves.”
Without human input to give context to the data AI can generate, brands will be unable to utilise the creative intelligence they have at their fingertips
Automating the platform’s processes ensures timely intelligence, which can help a business to take action. Drawing on the guidance and recommendations of AI at executive-level meetings removes hierarchy from the decision-making process. This could enhance agile thinking when critical actions are needed to outmanoeuvre opponents.
The evidence suggests that more businesses are using the technology. There are well over 10,000 roles for competitive intelligence advertised on LinkedIn, for instance. The global market size of competitive intelligence tools is projected to hit $82bn (£68bn) by 2027, according to research from Fortune Business Insights.
A significant proportion of this spend is likely to come from corporations whose deeper pockets allow them to deploy state-of-the-art large language models (LLMs) like GPT-3, which can provide more sophisticated data insights. GPT-3 is one of the most straightforward models for building CI. But it needs infrastructure, skills and software to work effectively, which don’t come cheap.
High-quality baseline data is essential
Regardless of the computational power of the AI model, it needs targeted and verified data to function and benefit a company’s CI strategy. That data needs to be drawn from multiple sources to stop its ‘intellect’ from becoming circular and repetitive.
AI models are only as intelligent as the training data they are given. The more data they’re trained on, the more accurate, versatile and useful they are to a business. This means there must be skilled oversight of the quality of the data.
“Training with low-quality data, like social-media posts or blogs, can introduce more noise into the learning process, which can confuse the model and decrease the quality of the outputs,” explains Kalyan Veeramachaneni, a principal research scientist at the MIT Institute for Data, Systems and Society.
Data bias can also be a consequence of working with low-quality data. For example, if data is limited to text from a particular group it can skew the AI’s function, lower overall performance and reduce the reliability of the intelligence.
But by monitoring data quality from sources with editorial filters or filtered web content and not relying too heavily on user-generated content, companies can increase the quality of the inputs, which will also raise the level of intelligence that they are receiving, in terms of reliability and usefulness.
Don’t lose sight of the human factor
Whether you’re basing your CI strategy on insights from an advanced LLM or a more ‘cookie-cutter’ SaaS platform, you will still need the human touch. Then you are matching competitive intelligence with creative intelligence from in-house teams to maximise any advantages and make the business stand out from the crowd.
“AI can be an asset for brands to stay ahead of the game in competitive markets, by increasing efficiency and insight within marketing teams and reducing time-consuming, laborious processes,” reveals Anthony Lamy, vice-president, client partnerships at VidMob. “However, without human input to give context to the data AI can generate, brands will be unable to utilise the creative intelligence they have at their fingertips.”
Businesses can therefore find a competitive edge, not by relinquishing complete control to AI, but by using it in tandem with human creative teams to build their creative intelligence.
So, it seems that whereas previously there was either huge distrust or blind faith in what the technology could achieve for an organisation’s CI strategy, there is now a realisation that if you’re not seriously considering investment in or scaling up AI-driven CI, it’s very likely that your competition will be.
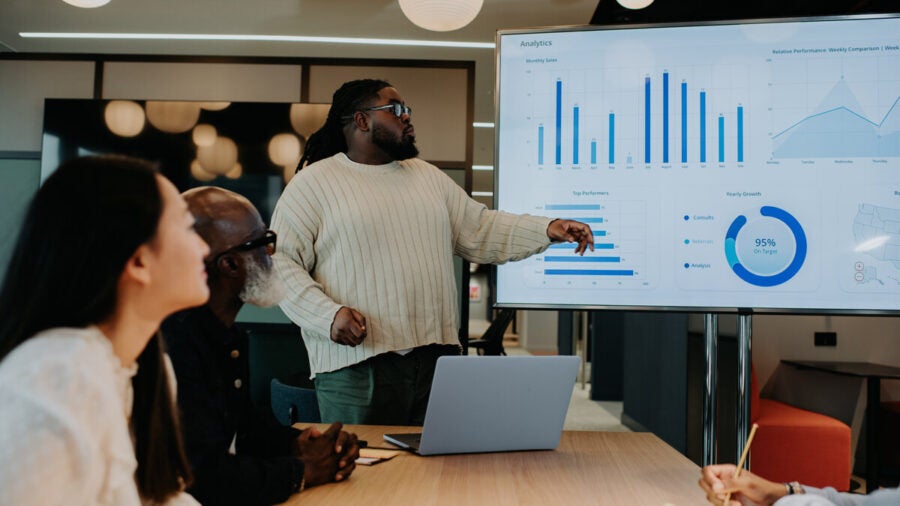
The business landscape is strewn with the corpses of corporations that failed to notice what competitors were up to, with every decade throwing up a new, high-profile victim of the head-in-the-sand approach to market share.
Think of Blockbuster’s dismissive attitude towards Netflix in the noughties and then even Netflix’s lack of foresight in seeing the streaming wars on the horizon.
This is why organisations need robust competitive intelligence (CI) strategies: to ensure that they are not blind-sided by competitors or disruptive business models.