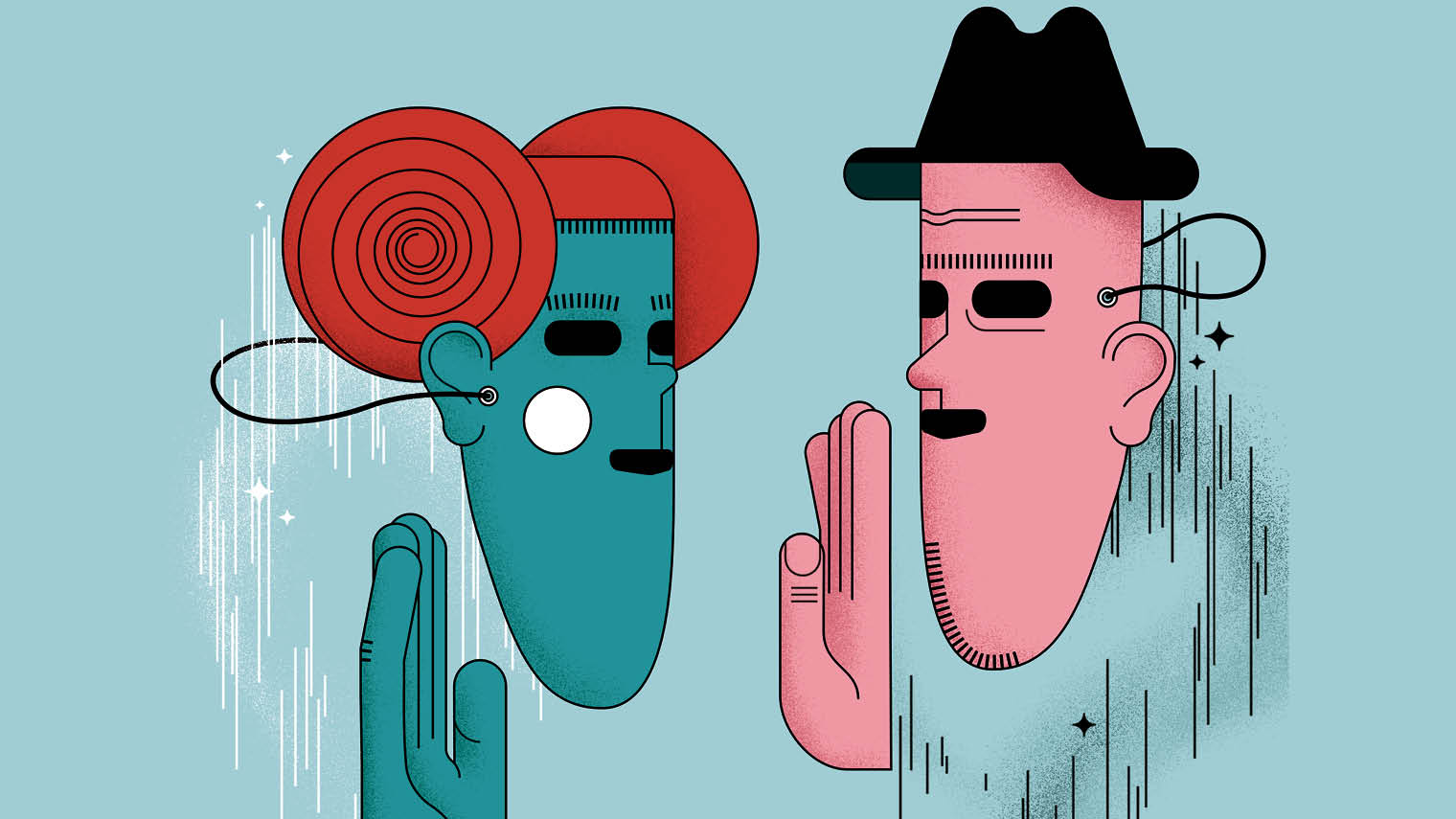
Most of us have seen a deepfake video at one time or another, be it Donald Trump appearing on Better Call Saul or Tom Cruise performing magic tricks on TikTok. The media coverage is often negative: from sexploitation to corporate blackmail, we’re constantly told that deepfakes will enable fraud and deception on a massive scale.
At American Express, however, the technology behind deepfakes is serving the opposite end: fighting fraud. By using hyper-realistic data to help train the company’s own detection systems, the company’s researchers believe they can warn customers more accurately and minimise the number of unnecessary card stoppages.
It’s certainly a bold strategy, not to mention a timely one. Global payment card fraud losses reached $28.65 billion in 2019, according to the Nilson Report. It’s almost certain this figure has risen during the Covid-19 pandemic; various financial agencies have reported an uptick in fraud during recent months, driven by a rise in online shopping.