Data is now king of the modern enterprise – is yours ready for its elevated role?
Businesses must tackle quality, governance and infrastructure gaps to unlock AI’s full potential
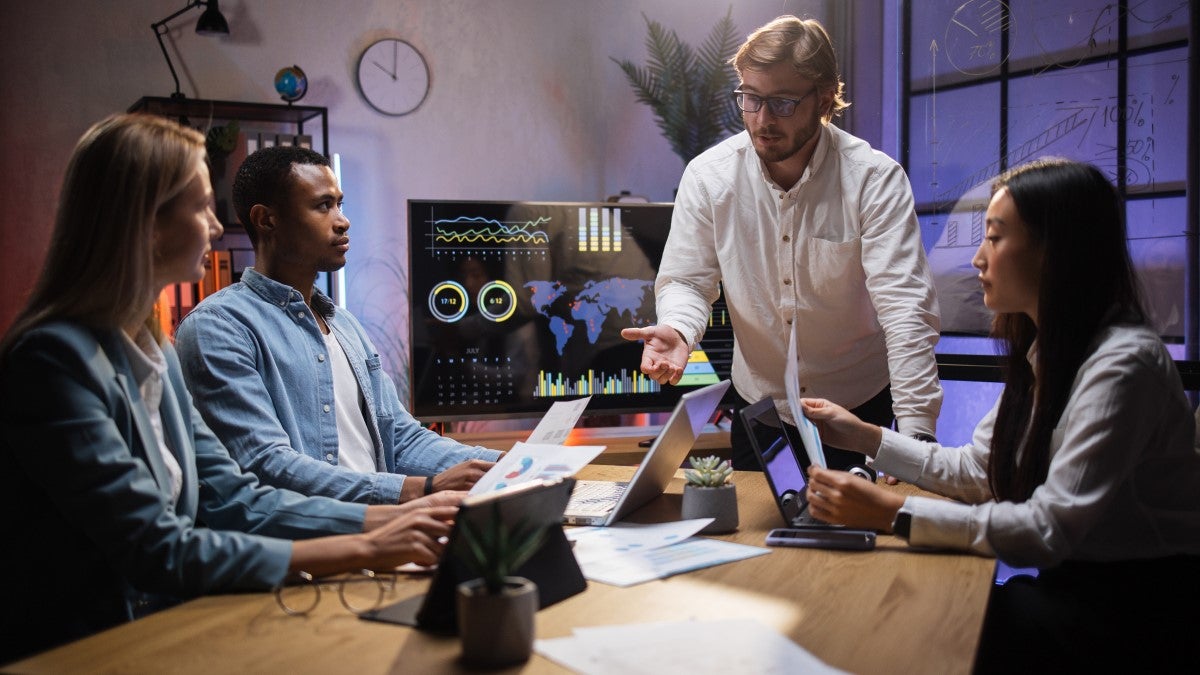
There is unrivalled enthusiasm among business leaders as to how artificial intelligence (AI) will impact their operations. The race is on to deploy AI applications at scale and take advantage of newer developments, such as agentic AI, in a bid to stay one step ahead of the competition.
However, AI is only as reliable as the data it learns from. Research shows that just 11% of CIOs have fully implemented AI, citing security and data infrastructure as top blockers.
A separate CDO study reports that only 38% of generative AI pilots have successfully transitioned into production. For 43% of data leaders, the quality, completeness and readiness of data is a key obstacle to success. They struggle with data inadequacies – particularly gaps in the data – and access to the data.
“One of the biggest hurdles in scaling AI is ensuring the integrity and quality of the data it relies on. If the data is incomplete, inconsistent, or biased, the AI outputs will be equally flawed, potentially leading to inaccurate decisions,” says Alana Muir, head of cyber at Hiscox.
A business issue, not an IT issue
The impact of poor data quality can be significant: some estimates suggest businesses can experience revenue losses of 6% due to inaccurate, incomplete and low-quality data feeding their AI models.
This, more than anything, demonstrates that data readiness is a business issue, not an IT issue.
“I’ve talked to a lot of organisations about data quality, and the worst statement I’ve heard is that data quality is an IT problem. It is not. Everybody in the organisation needs to work together to resolve those data quality problems,” says Dimitris Perdikou, chief engineer for the UK Government.
“If you don’t get the data quality right, even once you feed it into an AI model, you’re going to get a very poor output based on that data quality,” he told attendees at a recent AI in government event.
Businesses must therefore make sure their AI systems have access to the most accurate and relevant information to ensure teams and customers can trust the data. Dealing with data organisation issues, governance gaps and security vulnerabilities is more pressing than ever.
“As AI continues to evolve rapidly, businesses must establish clear, up-to-date governance policies. These should define how data is collected, stored, accessed and used across the organisation to ensure consistency, accountability and regulatory compliance,” says Muir.
How to ensure your data supports next-gen AI
So how can data leaders ensure their data infrastructure is equipped to support the next generation of AI, particularly in terms of handling the increasing complexity and volume of data?
It boils down to a few key areas, says Ingrid Verschuren, executive vice-president of data and AI and general manager for EMEA at Dow Jones.
First up is modernising data. “Outdated legacy systems often struggle to handle the scale and speed AI demands. Moving to more flexible, cloud-based architectures can provide the scalability and agility needed to support complex workloads,” she says.
Second, data quality must be a priority. “Cleaning, validating and standardising data ensures that models are being trained on reliable inputs. Without that foundation, even the most advanced AI tools will fall short,” says Verschuren.
Leaders also need to foster a forward-looking mindset. That includes upskilling their teams and encouraging them to explore the use of AI tools in their day-to-day roles.
Keeping a human in the loop
Elsewhere, the rise of agentic AI means organisations need to balance the potential for automation with the need to maintain human oversight.
“Agentic AI is already making waves – its ability to adapt to changing environments and quickly make decisions is impressive,” says Jo Drake, CIO at THG Ingenuity. “Human oversight is critical in the governance of AI in terms of enhancing system accuracy and safety and fostering trust in the technology.”
Muir also believes that no matter how advanced AI becomes, it can still make mistakes, particularly when relying on flawed data.
“AI-generated outputs should always be subject to human review to prevent costly errors,” she says. “The human-in-the-loop approach ensures experts validate AI outputs before they influence critical decisions. Rather than handing control entirely to AI, companies should implement human oversight at key touchpoints, maintaining accuracy and reliability.”
Building a successful data governance framework
Shockingly, 95% of businesses reportedly lack a comprehensive governance framework for gen AI. Organisations must therefore find a balance between building a robust AI governance framework – that ensures compliance with data privacy regulations – and fostering innovation.
Dow Jones has built its AI governance framework around speed, transparency and trust, says Verschuren. “Our AI steering committee is deliberately small, agile and cross-functional. This structure allows us to move quickly while ensuring every decision reflects the needs and perspectives of the wider business,” she explains.
“Crucially, this isn't a closed group. Our framework ensures that anyone at any level can raise ideas or concerns with the steer committee. That openness builds confidence – employees know that there’s a real team of experts behind every decision, not a black box.
“We also work in lockstep with our legal and compliance teams to protect our intellectual property and uphold the highest standards of data privacy. As a publisher first, we’re acutely aware of the value of our content and the importance of using data ethically. We can only spark innovation when it’s grounded in trust.”
Ultimately, says Muir, organisations shouldn’t view AI as a shortcut, but an amplifier.
“If businesses have strong data and security foundations, AI can enhance operations. But if weaknesses exist, AI will magnify them. The most important step businesses can take right now is getting their data in order and fostering a culture that values security and transparency.”
Agentic AI: how it works and how to make it work for you
AI agents are poised to transform business operations, but understanding their capabilities and ensuring robust data governance are crucial for successful implementation
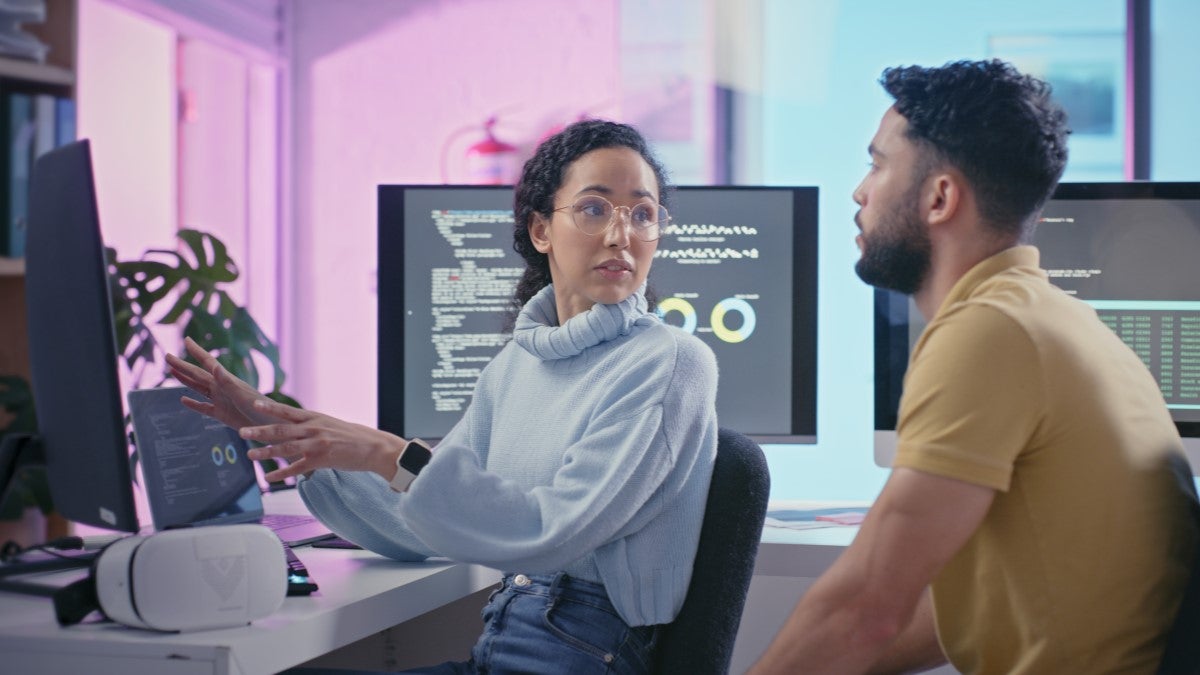
In the rapidly evolving landscape of artificial intelligence, agentic AI – or AI with agency – is emerging as a transformative force in business. Unlike traditional AI systems that rely on user prompts or operate within tightly controlled parameters, agentic AI is designed to operate more independently. It can perceive its environment, make decisions, and take actions based on real-time data – all with limited or no human intervention.
This level of autonomy enables agentic AI to complete multi-step tasks, dynamically adjust to new inputs and learn from its interactions over time. The implications are vast: streamlining workflows, accelerating decision-making and amplifying productivity.
According to Gartner, by 2028, a third of enterprise software applications will include agentic AI – up from less than 1% in 2024. Gartner also suggests that it will enable 15% of day-to-day work decisions to be made autonomously.
But to unlock this value, businesses must understand that agentic AI isn’t about wholesale automation, but the strategic augmentation of human potential.
“The real value of agentic AI is that it allows you to offload more of the work that isn’t necessarily creative, thoughtful, critical thinking work – but the work that increases efficiency and effectiveness,” explains Marla Hay, vice-president of product management at Salesforce. “It enables a business to really increase its efficiency and alleviate hours of work.”
A new way of working with AI
Agentic AI is already reducing development time in areas such as software engineering. Developers can create code faster by working in conjunction with AI assistants.
“You’ve got a person operating in conjunction with an AI tool, and they can say things like, ‘Hey, can you create XYZ?’ – it spins it up, and then you can make adjustments, iterating on the output. That’s one part of the spectrum,” says Hay.
Salesforce has customers using AI in diverse ways to enhance operations. For example, Adecco is using AI to make the application process easier for candidates. Adecco can use AI on two counts: matching employers with employees, and positions to people.
“We’re seeing it increase efficiency dramatically across all areas of our customers’ businesses. And that’s why there’s such a huge opportunity here,” says Hay. “It enhances the existing capabilities of the workforce – and entire industries – in ways we haven’t seen before.”
Data as the foundation
Despite the promise, just like any other type of AI, agentic AI is only as effective as the data that powers it. Organisations need to focus on building robust, real-time data infrastructure to make the most of this technology.
“At the end of the day, it’s all about the data,” says Hay. “Agents can’t materialise answers without having that foundation of data sitting underneath – data that’s accurate, reliable and actually enables the agent to create efficiencies in the system. It all starts with data.”
This begins with ensuring the data exists in usable form: it must be backed up, available and recoverable. Next is accuracy – clean, standardised data is critical, particularly as AI models can easily be thrown off by inconsistencies.
“Sometimes it’s duplicative data that’s just slightly off, or old data that’s no longer correct, but the AI is still using all of it to make decisions. So, if you’ve got old or irrelevant data in there that you wouldn’t want the AI to use, you should be pulling it out,” says Hay. “Having a data lifecycle plan that includes archiving is really important to make sure the data your AI is using is accurate, so your tooling can generate answers in a reliable way.”
But while there’s a lot of hype – and hope – around AI, only 11% of CIOs have fully implemented the technology, with hesitation stemming from concerns around security and governance.
Hay stresses that organisations need clear frameworks to govern how data is accessed and used by AI agents.
“A key aspect is making sure you have clear data permissions, that you know exactly who can access what, that those permissions are kept up to date and that your agent has the same controls,” says Hay. “You’ve got to make sure your agent can only access the data it should, and not the data it shouldn’t. That’s foundational.”
Data masking and privacy safeguards are also essential. When training or testing agents in sandbox environments, personally identifiable information (PII) should be excluded or tokenised.
“It really comes down to this: make sure your AI has access to the right data – and then make sure you can understand what it’s doing with that data,” says Hay.
Culture, trust and transparency
Alongside technology and data, preparing people and processes is vital. Salesforce, for instance, relies on an internal ethics board to review AI use cases from multiple perspectives.
“Start to finish, everything we’ve done with AI has started with that board,” says Hay. She adds that it’s really important for leaders to ask themselves how they can make sure they’re doing the right thing and how it could go wrong when implementing any new capability.
“We’ve had our trust, compliance and privacy teams riding alongside everything, making sure we’re not just staying compliant with laws but also doing what our users and our customers would expect from us.
“That’s the goal: if someone’s interacting with AI, they should know it’s AI, and they should know that what’s coming back is being validated to be non-prejudicial, fair, accurate – and that someone is actively making sure it’s working the way it’s supposed to.”
Even if an organisation doesn’t have an ethics board, leaders should at least consider having a cross-functional team in place. That might include CISOs, CIOs, legal, trust and compliance leaders working together on how they’re going to approach this new era of AI. This collaborative approach helps ensure transparency, trustworthiness and alignment with emerging regulatory frameworks like the EU AI Act.
“Data is at the heart of AI, and if you can get the data right, you’re going to be off on a great foot. Data accuracy, data reliability and the ability for an agent to use data effectively are the cornerstones of making this work – and making it work well,” says Hay.
“Agentic AI is such a huge revolution and will be so beneficial to everyone involved. So how do we do that in a way that makes people comfortable and makes sure that we’re doing it with guardrails that allow us to grow and mature?”
The AI-ready workforce: skills every business needs in the digital age
Data and AI skills are in high demand, but organisations must think carefully about their exact needs and invest in upskilling to build future‑ready teams
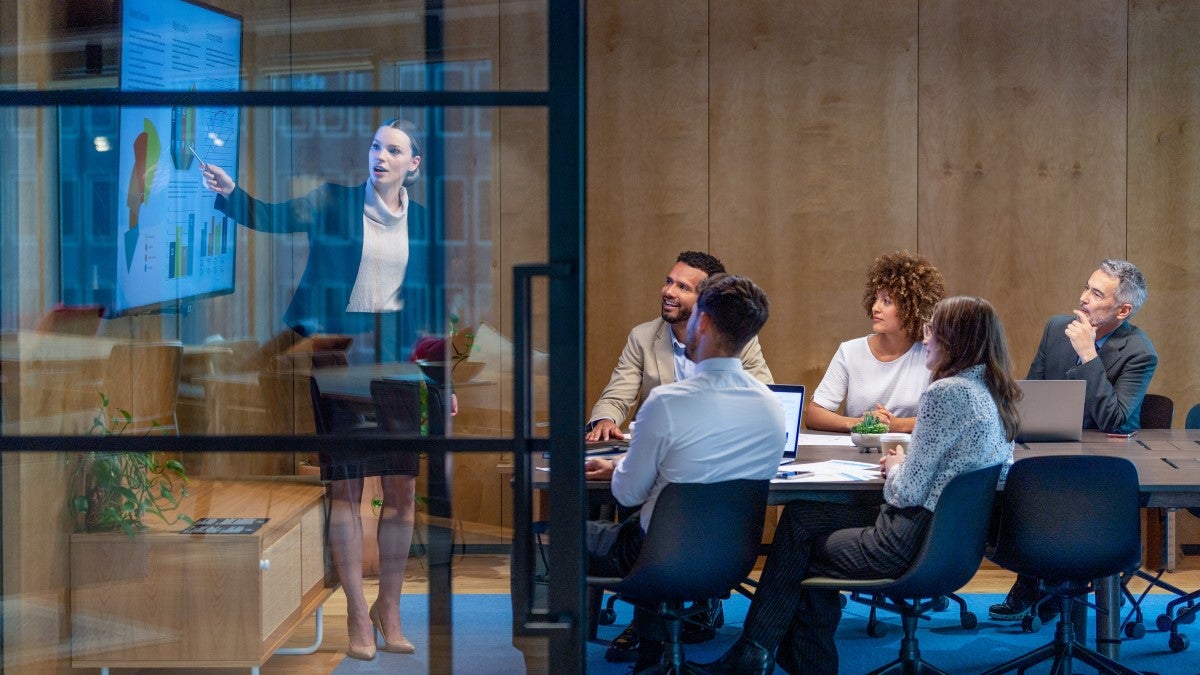
Data and AI capabilities are in more demand than ever, topping the list of fastest-growing skills in the World Economic Forum’s 2025 Future of Jobs report.
But with AI and big data skills topping their wish lists, how can companies attract and retain the talent necessary to stay competitive in this evolving landscape? It’s something that enterprises are grappling with, especially if they want to take advantage of developments such as agentic AI.
Antony Bradshaw, head of digital analytics at Domino’s Pizza UK, maintains that sustaining an environment of “technological excellence and meaningful innovation” is the most effective way to attract AI and data science professionals and foster long-term engagement and loyalty.
“The most talented professionals in AI and data are attracted to challenging work that keeps them at the forefront of innovation,” says Bradshaw. “Investing in the latest tools, technologies and platforms is key to retaining them. Leading talent in AI and data seek exciting projects with real-world impact that challenges them and support their growth, rather than wrestling with antiquated infrastructure and architecture.”
Upskilling is a key component of AI strategy
Elsewhere, the national mapping agency for Great Britain, Ordnance Survey, is including upskilling as a key component of its AI strategy, to ensure it manages the impact and risks before adoption. This is supported by an internal change management team.
The human element remains a critical factor, says Ordnance Survey CTO, Manish Jethwa.
“While advanced digital tools powered by generative AI may pass the Turing test, we need to be careful that, in our aim to enhance efficiency, we don’t lose the personality, creativity and emotion that we bring as humans into the workplace,” says Jethwa.
“This is crucial to building strong relationships with our customers and partners but also key for collaboration and teamwork that defines the business culture and enables it to evolve to meet unforeseen challenges.”
Jethwa says executives and managers need to set an example by demonstrating how they are using the technology. This helps alleviate any concerns or doubts about using AI and demonstrates that leveraging tech is a legitimate and beneficial practice.
“The rapid developments in AI require a critical mass across the organisation to track these advancements and ensure everyone keeps up. Building upskilling goals into personal development plans can be an effective strategy to demonstrate your support of employees in allocating time to AI training,” he says.
Democratising data across the workforce
This rapid evolution means businesses have no choice but to address the cultural and organisational shifts needed to integrate AI into the workforce. However, Jack Berkowitz, chief data officer at Securiti, challenges the notion that every company must become an AI powerhouse.
“Do you actually need the PhDs that can devise the algorithms or train the model, or do you need the application development team that can take advantage of somebody else's technology?” he asks.
Berkowitz advises organisations to “focus on your core competency and outsource the rest.” This means companies should prioritise understanding how AI can solve their specific business problems.
At the same time, every department needs to be data-savvy enough to support AI-driven workflows and decision-making. More than a fifth of organisations currently using GenAI say they have fundamentally redesigned at least some workflows, according to McKinsey.
So, it’s important that enterprises ensure employees are proficient with company-approved AI tools – and are avoiding the risks associated with unsanctioned AI technology.
For its part, Domino’s has “democratised data” within its organisation, making it easily accessible to business stakeholders and franchise partners through dashboarding tools. The company has also implemented an AI council comprised of IT, legal and data professionals, whose focus is to understand the usage and requirements of AI tools within the workplace, says Bradshaw.
“This council enables us to have a balanced approach to implementing and assessing new tools allowing upskilling in new software and trends, while also restricting access to higher risk software,” he says.
“To supplement our more structured learning, we have multiple internal communities to allow bright minds to come together and discuss their work, such as our analytics community which sees data professionals across the business bring specific problems or pieces of work for support or critique.”
Don’t underestimate your workforce
At a high level, Berkowitz challenges the belief that employees struggle with technology. He points out that most employees are already sophisticated tech users in their personal lives.
“You’re on Instagram every day, on Spotify and Netflix,” he says. “And guess what? Those are data-driven systems, just the same way as these enterprise systems.”
The barrier, he suggests, is not employee capability but organisational perception. Berkowitz is critical of companies that underestimate their workforce. “This notion that ‘my people can’t possibly do that.’ Well, why not? Appreciate your people because they’re able to do it.”
Aligning AI and business strategies
As businesses navigate the complex landscape of AI adoption, they should align their AI strategy with broader business strategies – and make the strategy accessible to all employees. Says Jethwa: “This ensures that everyone is on the same page and working towards common goals.”
And as a data leader, that means empowering, facilitating, and supporting the business’s ability to make data-driven decisions, bridging the gap between the data, users, management, and the organisation.