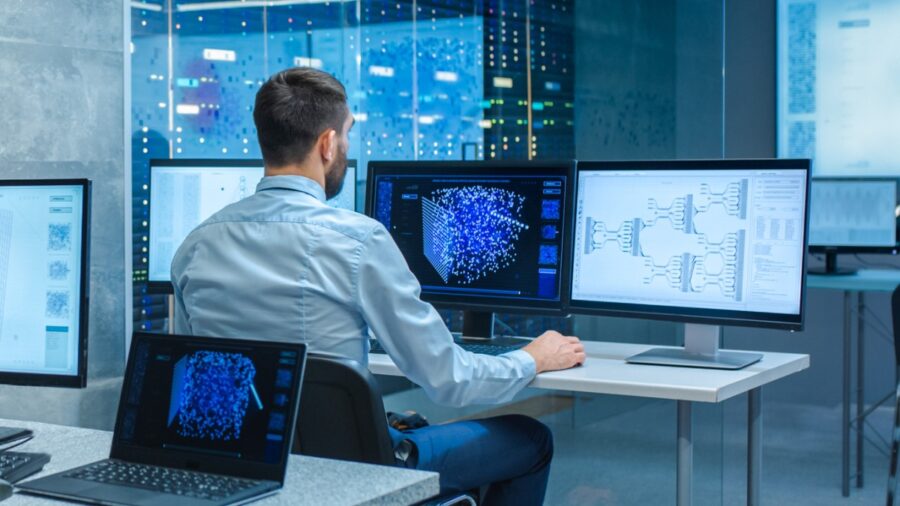
Many organisations are still at the stage of small-scale generative AI experimentation. For example, they might be trialling it as a tool to improve individual productivity or streamline team processes. But businesses that balance immediate improvements with bigger and longer-term thinking will likely come out on top.
According to Deloitte’s 2024 Q2 State of Generative AI report, organisations with a good grasp of the technology are already thinking big. The survey found that 63% of organisations with very high generative AI expertise see driving innovation and growth as a key benefit of the technology, compared to 35% of organisations overall. So, what do four business and technology leaders have to say about innovation potential in this space?
We don’t know exactly where a lot of this is going but large language models (LLMs) will continue to evolve. And you’ll likely need more than one depending on what problem you’re trying to solve or what opportunity you’re going after.
I’m also starting to hear more forward-looking conversations around rethinking how organisations do business and how they engage customers. What are the implications for doing that at scale? Scaling AI experiments will require a combination of technology maturity, skills and changes to ways of working. In the UK alone, if we get the foundations right, there’s a £109bn opportunity with AI and machine learning through to 2030.
The opportunities in healthcare in particular are immense. Places like the Allen Institute are developing the first full brain map. Just imagine what that could mean in terms of the diagnosis of some diseases. And the generation of things like synthetic data could help run drug trials faster. In those areas where you can really help people, both from a wealth point of view and a health point of view, it will be a game changer if everyone comes along on this journey.
What does this mean for businesses now? We often talk about what’s going to change and what isn’t going to change. The need to get cloud and data foundations right isn’t going to change, nor is the fact that we’ll be asked to experiment faster and be more agile. So start working on those mechanisms that give you that agility to experiment faster.
I think we’re going to stop dividing this concept of a tech function and a business function. Our chief data officers are amazing business people. This idea that there’s a dichotomy between the two skills is rubbish. We’re going to see more and more people coming through with these wonderful mixed profiles, and that’s going to be transformative.
If I were looking in my crystal ball, there are a few things I’d be keeping an eye on. One is that ESG reporting is coming in at the same time as GenAI, so the environmental responsibility of these new models needs to be addressed. There’s also a discussion around open-source large language models that anybody can improve or adopt, but they’re also easy to corrupt.
We’ve got some amazing use cases within specific sectors. If you want to see where this is going positively, look at the life sciences, they’re doing amazing work. They’ve understood their needs and how to harness these technologies within their existing framework. It’s a field that knows how to make the most of the technology. They were ready. They were waiting. They had problems they knew they urgently needed to solve. They had a mission.
And then there are some other things we’ve got coming on the horizon like interactive AI, where these incredibly narrow AIs start talking to each other. So your cancer detection for skin cancer starts talking to the detection for spine cancer, and they start working out together which is more likely. Whereas at the moment, you need two completely separate AIs for those skills. So there’s a lot of investment in research in that area at the moment.
All organisations using AI will need to think about who is in control. You probably want both machines and humans working together but how you delegate responsibility will depend on the use case.
If you look at GenAI and where it will go in the future, it’s not just a business thing. It’s enabling new forms of creativity and expression. There will probably be a new sector or a new form of art that we can’t see yet enabled by AI.
We’ll probably see wearable health tech becoming much more intelligent. So you don’t have to work it out when you think: ‘Oh, God, I’m a little bit hotter today. What does that mean?’ It’ll calm a load of people down when there’s intelligence behind that to say you’re fine, you’re just a little bit run down.’
It’s an incredibly exciting space and I’m very much looking forward to the future, but we’ve always got to control ourselves with the ethical, social, authenticity and ownership questions.
Right now, businesses should be working out what they want AI to fix. What is your business problem that you want AI to solve? Work that out first before jumping into it.
Short term, I think getting GenAI solutions to scale will be the main theme. After a year of largely experimentation, the focus for many will shift to wanting to know what the concrete benefits are from a business perspective. We may see some impatience in seeing results, and overall a trend to sharpen the use cases and value propositions where GenAI plays a role.
Then we’re going to see the next wave of LLMs. There are a few recent announcements and more on the horizon, but the question is what comes after that? From a compute and data perspective, what does a sustainable operating model look like? At what point do you need to start branching out into more specific models (like models tailored for life sciences or, in our case, to financial services)? How do you find that balance between general and more niche models? Or will mainstream models become so good you don’t even need these? Becoming more specific will be next from a technology perspective as it will drive the quality of outputs. With such a high pace of innovation, the future is hard to predict and we will need to revisit assumptions frequently.
Organisations should reflect this uncertainty in their adoption strategies and ensure they can pivot and protect investments at any time. Many will rely to a different degree on technology partners to deliver their vision, so they must ensure these partners can support them on this journey.
Find out more about how AWS can help you on your GenAI journey
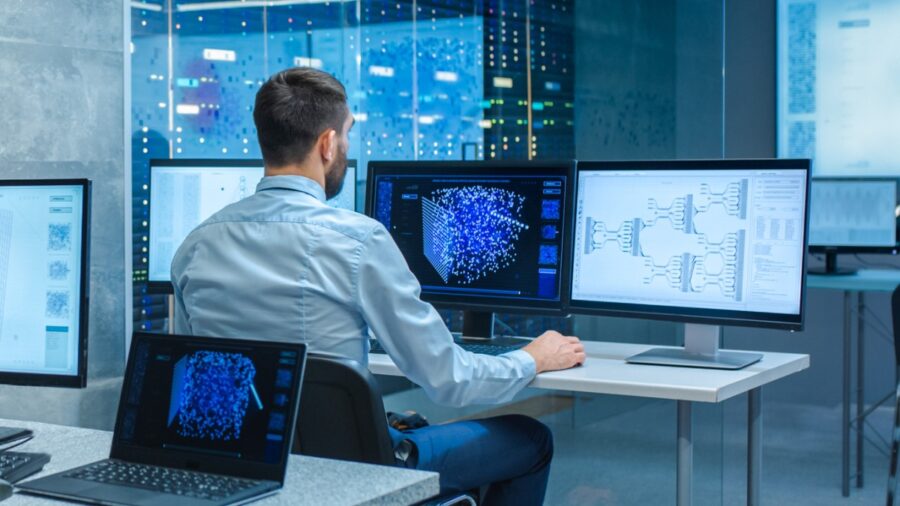
Many organisations are still at the stage of small-scale generative AI experimentation. For example, they might be trialling it as a tool to improve individual productivity or streamline team processes. But businesses that balance immediate improvements with bigger and longer-term thinking will likely come out on top.
According to Deloitte’s 2024 Q2 State of Generative AI report, organisations with a good grasp of the technology are already thinking big. The survey found that 63% of organisations with very high generative AI expertise see driving innovation and growth as a key benefit of the technology, compared to 35% of organisations overall. So, what do four business and technology leaders have to say about innovation potential in this space?
We don't know exactly where a lot of this is going but large language models (LLMs) will continue to evolve. And you'll likely need more than one depending on what problem you're trying to solve or what opportunity you’re going after.