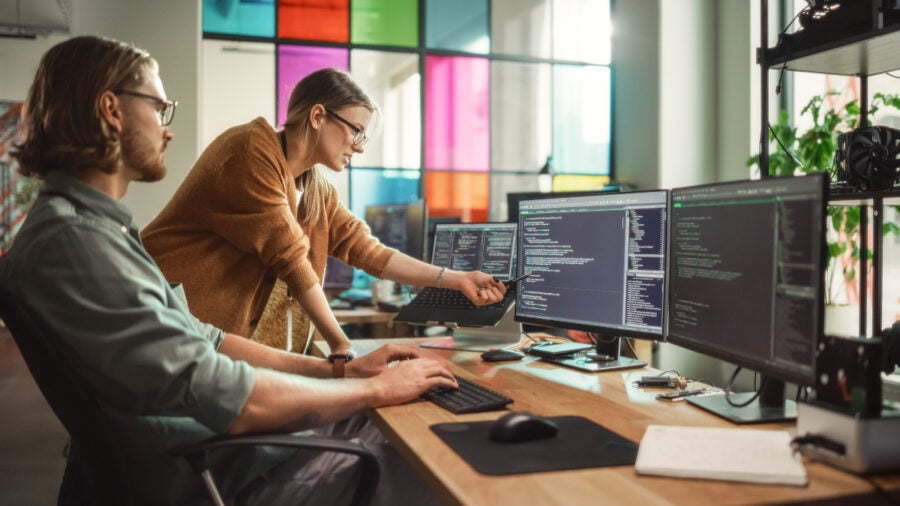
There’s an old saying in the culinary world: never trust a skinny chef. If they aren’t eating their own food, why should you?
The same logic applies to AI companies. If you’re not using your own product day-in, day-out, how can you truly understand what works and – more importantly – what doesn’t?
Too often, AI companies build tools that look impressive in a controlled environment but fail in real-world use. This happens because companies prioritise cutting-edge features over usability. They chase the next breakthrough rather than focusing on what their end users actually need. But an AI model that isn’t tested in the chaos of real-world workflows is like a beautifully plated dish that tastes awful – all style, no substance.
The best AI players are doing things differently. They embed their own technology into their daily operations, test it rigorously and refine it based on actual user pain points. They break their systems before their customers do, facing flaws and quirks head-on to build a genuinely helpful product.
Eat your own AI
It’s easy to fall into the trap of designing AI in a vacuum. Part of the problem is the culture around AI development, which has been defined by an obsession with scale-bigger models, more compute power, and ever-growing infrastructure.
But this was never going to be sustainable. We are already seeing signs of a shift. In recent weeks, Microsoft reportedly pulled back on data-centre leases, a possible sign that even the biggest players are re-evaluating whether bigger is really better.
Raw power does not fix fundamental usability problems. Building big shiny solutions without integrating them into real-world workflows creates a dangerous blind spot. AI models can be fine-tuned to perfection in controlled testing environments, but real-world applications are messy. User inputs are unpredictable, data quality varies, and assumptions made in development often collapse when tested in the wild.
Some have it easier than others
Of course, not every AI company builds technology that naturally fits into its own business. If you’re working on AI for fraud detection, legal analysis, or niche areas of manufacturing, your internal team may not be the target user. But that just means you need to be more deliberate about testing.
If direct use isn’t an option, create structured real-world scenarios. Set up internal sandboxes where AI is tested under the same conditions customers will experience. Bring in industry professionals to work alongside your team – not just as testers, but as embedded users providing continuous feedback.
Another approach is shadowing. Engineers shouldn’t just look at AI outputs. They should also sit with real users and observe how they interact with the system. What workarounds do they invent? Where do they hesitate? What breaks their trust in the AI’s output? These details rarely show up in standard testing, but they determine whether a product succeeds or fails in practice.
Three things every AI company should do before they release their product
It’s easy to incorrectly assume an AI system is working well if you’re only looking at outputs. But what about the experience of using it? Ask yourself:
- Does it introduce unnecessary complexity?
- Does it take too long to generate useful results?
- Do the outputs feel predictable, generic, or off the mark?
The worst time to discover an AI failure is when a customer is already relying on it. Before that happens, figure out where it hallucinates and what types of data cause unexpected errors.
Does it generalise well across different datasets, or does it fail outside of its training parameters?
One of the biggest misconceptions in AI is that it should be used everywhere. In reality, AI has clear strengths and weaknesses. Companies need to be realistic about where their products add value.
If a customer has bought into the hype and is expecting too much, push back. Start small. Focus on simple, high-value use cases. Avoid grand promises and let the technology prove itself by delivering real impact.
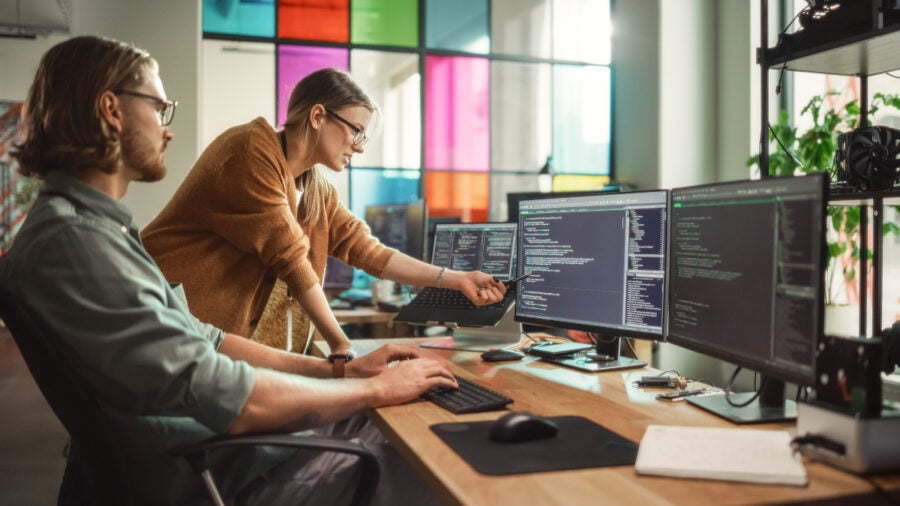
There’s an old saying in the culinary world: never trust a skinny chef. If they aren’t eating their own food, why should you?
The same logic applies to AI companies. If you’re not using your own product day-in, day-out, how can you truly understand what works and – more importantly – what doesn’t?
Too often, AI companies build tools that look impressive in a controlled environment but fail in real-world use. This happens because companies prioritise cutting-edge features over usability. They chase the next breakthrough rather than focusing on what their end users actually need. But an AI model that isn’t tested in the chaos of real-world workflows is like a beautifully plated dish that tastes awful – all style, no substance.