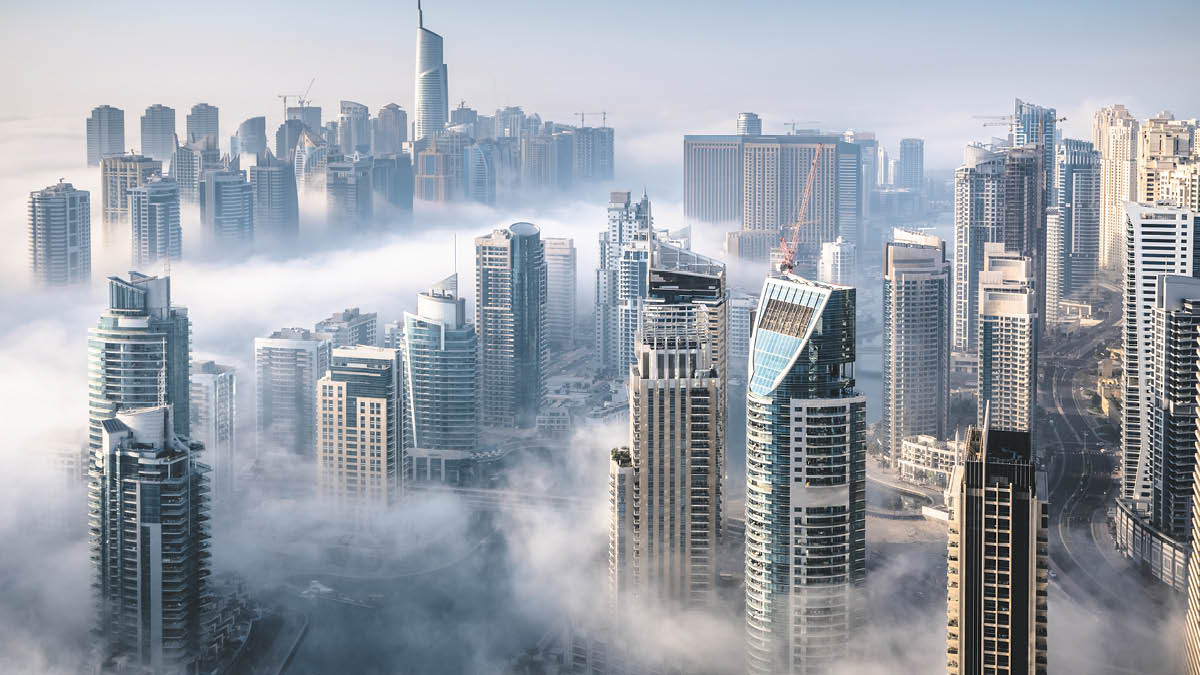
There is no doubt that the potential of AI is exciting. Whether it’s driverless cars or faster drug discovery, AI is changing our world and how we live in it.
In the corporate world, financial analysts are able to use AI to improve the quality of their market analysis. Using AI, organisations can pull together and analyse millions of pieces of data from company tweets to regulatory filings and even media appearances, to identify patterns of language and behaviour. In other words, AI can tell you whether a company’s good news is really good news.
Still, despite the exciting and futuristic applications of AI technology, many early adopters have struggled to deliver any ROI or business value outside of small, experimental projects. Gartner reports that, in 2022, 48% of CIOs have already deployed or plan to deploy AI and machine learning technologies in the next 12 months. However, they also report that many of these organisations won’t be reliably using AI in production until 2025.
The problem isn’t usually with AI itself, but rather with delivering AI at scale, says James Coomer, vice-president for product management at DDN, which specialises in storage systems for AI. Having solved the scalability challenge for some of the largest global AI projects, DDN now helps organisations plan their AI journey to production. These challenges are more often linked to the large-scale implementation and deployment of AI, as organisations look to deliver greater value and innovation to their customers and shareholders.
For example, some use cases like online retail and consumer preference analysis can work well in modest deployments, using datasets that are not too large in size. On the other hand, natural language processing and real-time video analysis need datasets that are well beyond the experience of traditional IT teams.
“Truly delivering the benefits of AI means dealing with volumes of data that are orders of magnitude higher than anything we’ve seen before,” says Coomer. “Not only is the volume of data higher, but much of this data is rich, unstructured information like video, audio or images.”
For some organisations, the ability to gather and process very large volumes of data can unlock the next level of innovation. For example, DDN recently worked with Recursion Pharmaceuticals to use AI to accelerate the process of drug discovery. “Using AI rather than conventional methods can massively speed up our ability to learn and develop new vaccines or medications,” says Coomer.
DDN has 20 years’ experience supporting customers’ AI projects across sectors such as financial services, automotive and life sciences. It doesn’t surprise Coomer that some experts report that 87% of AI projects never make it past the prototype stage. While projects are small, data volumes can be easily managed. But once a model is successful, it will accelerate, and the volume of data and rate of data ingestion will both increase rapidly. “It’s something that often takes people by surprise,” he says.
Despite this, Coomer is optimistic that AI is on the verge of throwing off its reputation for being complicated, costly and frequently unsuccessful. “What we are now seeing is that the market is becoming more mature, the tools are more mature, and it’s often possible to buy AI-optimised systems that make it much easier for organisations to run AI projects at scale,” he says.
Truly delivering the benefits of AI means dealing with volumes of data that are orders of magnitude higher than anything we’ve seen before
At the same time, we are building a better understanding of how to run AI projects to the same standards as other types of development, Coomer adds. DDN advises clients to consider adopting the ‘AI factory’ approach. This is an approach to AI projects that allows organisations to build a consistent framework of processes around people, data, processes and production that can be replicated, making it easier to scale AI projects in future.
If a traditional factory builds a large number of physical products in a cost-effective and reliable manner, then the AI factory does the same with AI solutions. An AI factory provides a framework for organisations to build large numbers of AI models reliably, and at scale. It is more than just MLOps (machine learning operations) – it combines this approach with DataOps, ModelOps and DevOps to create a consistent approach to accelerating
AI development.
An AI factory framework might define agreed processes and approaches to things like how security is applied to data, how the data platform is managed, or how silos are reduced to improve overall performance. “It’s a reference architecture that reduces the risk and work involved in building AI at scale,” explains Coomer.
By adopting standardised processes and governance, the risks associated with AI are massively reduced. For organisations that are new to AI, Coomer suggests thinking about AI projects in terms of four
essential requirements:
- First, you need to be able to identify what data is needed; what is the right data source and how will you capture and organise that data?
- Second, do you have appropriate governance in place? If you’re building an AI model that will make recommendations about insurance cover, how do you prove that the model is trained and using data reliably?
- Third, it’s important to have the right people with the right tools. To build knowledge, make it easier for data scientists and data teams to share knowledge and collaborate.
- Finally, ensure you have the right platform to process data efficiently. Many projects fail because of data bottlenecks or because your network and devices cannot process data at scale.
According to Coomer, many AI systems drown in data as soon as they move out into the real world because traditional approaches can’t manage the huge volumes needed to feed large-scale AI models. “AI systems need to ingest data at incredibly high rates, and you need to make that data accessible and secure to enable the kind of deep learning needed to make critical decisions.”
When it comes to the fourth requirement, Coomer advises companies to think carefully about the capacity of their storage systems. “In our experience, people think about AI projects in terms of GPU and computing requirements, but don’t consider the data ingestion, and the volume of data that will be involved once an AI model goes into production,” says Coomer. “You could have the best data scientists and models, but if your storage can’t ingest the 250 petabytes of data that’s supporting your AI model, then your project will fail.”
Businesses should prepare for much larger data requirements by adopting AI-optimised systems and processes. These are systems developed with AI in mind, so that adjustments are made at every stage of the data journey to improve the speed and efficiency of data ingestion and transfer. In its State of AI in the Enterprise report, Deloitte found that the top priority for IT leaders investing in AI was modernising the data infrastructure for AI.
These days, AI does not necessarily need to be the complex, painful learning curve that early adopters have travelled, says Coomer. Companies embarking on AI projects today have access to an increasingly large number of AI-optimised tools and platforms that make it easier and faster to deploy AI at scale. Deloitte found that 80% of technology leaders are buying at least as much AI technology as they build, and the longer a company has used AI, the more likely they are to buy commercial platforms and tools.
To achieve quick wins from AI, organisations must invest in all four pillars of AI – data, people, process and platform. “They’re all important, and what we focus on at DDN is solving the platform part of the issue, whether you need a twentieth of a single rack or something that can handle petabytes of data without increasing the complexity,” says Coomer. “The key thing to remember is not to fall into the trap of doing what you’ve always done, and rather start with something that’s built to solve the problem in front of you.”
Q&A: Unlocking the promise of AI
Alex Bouzari, CEO of DDN, talks about how enterprises can get their AI projects right
Who is DDN, and how do you help organisations on their AI journey?
DDN is a market-leading global organisation which provides powerful data storage solutions, and facilitates the AI- and data-enabled digital transformation journey for enterprises and research facilities. With over 10,000 customers and thousands of deployments worldwide, DDN is making it easier, safer and faster to implement AI and reap massive rewards in healthcare, autonomous driving, finance, retail and many other sectors.
We de-risk the customer journey to a successful AI transformation: AI systems need enormous amounts of data to unlock meaningful business value: at DDN, we accelerate their digital transformation journey, by delivering the fastest path to implementation of AI, helping them move seamlessly from prototype to production. This means that organisations can focus on business value, instead of managing technical complexity and having to make compromises on data and scale.
Many business leaders see AI as a daunting, complicated undertaking. Is that a fair assessment, and how are things changing?
I think when any new technology comes along that has the potential to transform how we do something, people get baffled. The market is maturing so rapidly and there are now so many more organisations who can help you to implement AI strategies without delay or complexity.
The important thing is to interview and select vendors and partners carefully. Have they done this before? Do they know your market? If you get the right people and they have the right experience, you will get success.
What are the key steps a CIO must start with to unlock business value and benefits from AI?
I firmly believe to succeed with AI you need the right infrastructure, and that means having platforms that can deal with the massive amount of data and then help you to find the virtual needle in that haystack.
People wasted a lot of time trying to get their existing infrastructure to support AI, but you need new data repositories that are optimised for AI, and they need to be integrated with the business. That integration process has matured significantly in recent years, and there are now many more bridges between AI platforms.
What’s the most exciting application of AI you’ve seen recently?
We recently helped build a number of AI systems to support critical pharmaceutical and life sciences research. This is an exciting growth area, where companies are using AI supercomputers to discover new types of therapies and to improve the design of antibodies to help treat inflammatory diseases such as asthma and COPD. Digital biology is attracting both established organisations and emerging startups because it changes the economics of drug discovery.
What would you say is the biggest mistake companies are making with AI right now?
I think it’s trying to use existing technology for new purposes. A lot of technology exists with the purpose of keeping the lights on, managing the database, and taking care of operations. It’s not often seen as an enabler. Now things have changed, because AI is a business enabler, and the volume and type of data required to support that enablement is completely different to what we’ve seen before. It’s not that it’s much harder, it just needs different tools and platforms. Don’t take my word for it. Do the research. Go and see the successful implementations, see who has delivered, and ask them how they achieved those better outcomes.
Over the next five years, what’s the biggest change we’ll see in how we approach AI projects?
I think within five years we will start to see customised implementation of AI tools and platforms because organisations will want AI that meets their specific needs. Beyond that, I’m excited to see AI used in intelligent homes. I don’t just mean automated smart lights or music, but things like medicine built into intelligent dwellings to enable us to live healthier and more active lives.
To find out more, visit ddn.com/ai-for-business
Promoted by DDN
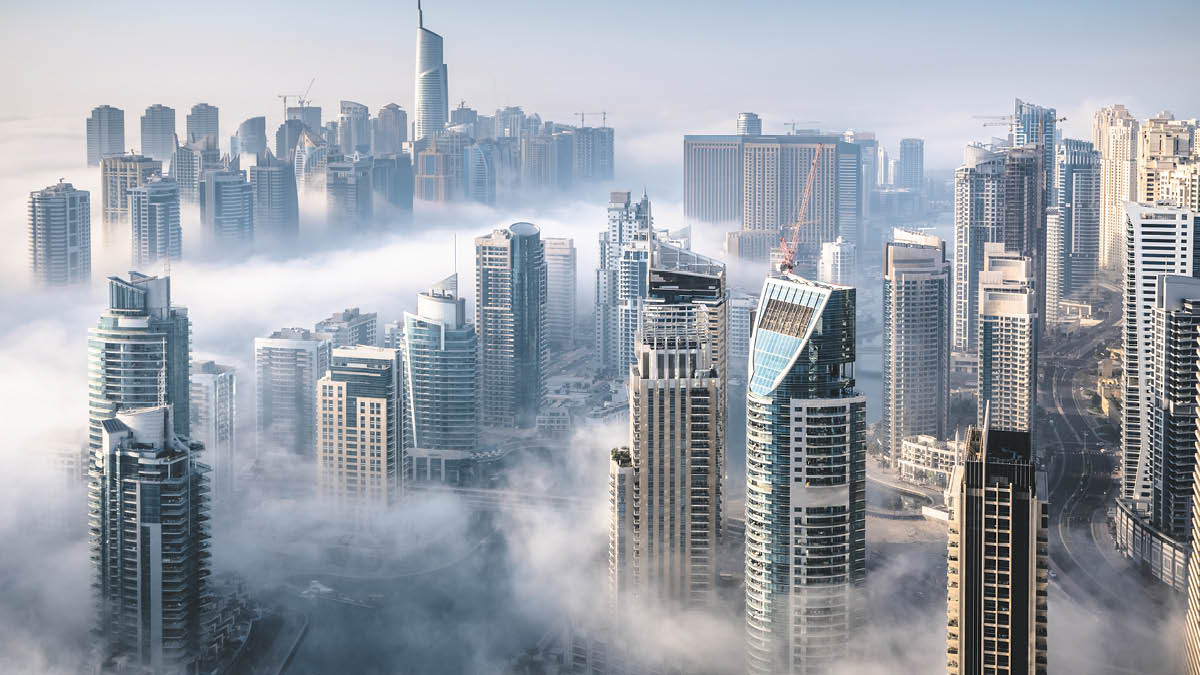
There is no doubt that the potential of AI is exciting. Whether it’s driverless cars or faster drug discovery, AI is changing our world and how we live in it.
In the corporate world, financial analysts are able to use AI to improve the quality of their market analysis. Using AI, organisations can pull together and analyse millions of pieces of data from company tweets to regulatory filings and even media appearances, to identify patterns of language and behaviour. In other words, AI can tell you whether a company’s good news is really good news.
Still, despite the exciting and futuristic applications of AI technology, many early adopters have struggled to deliver any ROI or business value outside of small, experimental projects. Gartner reports that, in 2022, 48% of CIOs have already deployed or plan to deploy AI and machine learning technologies in the next 12 months. However, they also report that many of these organisations won’t be reliably using AI in production until 2025.