When most people talk about big data they have a fuzzy sense of large quantities of digital material in mind. When Tim Barker talks about it, he knows exactly what it means. The chief product officer of software house DataSift sees his business devouring 40,000 items of data per second.
This prodigious appetite for information is triggered by DataSift’s core purpose. The company consumes information flowing out of social media sources such as Twitter and Facebook and turns this into a commercially viable product. The torrent of data is such that a raw social media feed is referred to as a firehose.
The sheer volume of data generated by social media presents a problem to market researchers. How can they isolate valuable nuggets of information from the mass of irrelevant messages? This is where DataSift, a four-year-old UK business that has already expanded into the United States and Canada, comes in. DataSift sorts and interprets the data until it becomes material that market researchers can make use of.
Mr Barker and his colleagues scour the outpourings from firehoses separating messages into topics such as food, fashion or finance. They divide their work between sentiment and entity analysis. Sentiment analysis attempts to define the emotion behind a comment, spotting irony or sarcasm. Entity analysis spots the meaning behind a word, such as whether “apple” refers to the fruit or the computer company. Both of these tasks are inherently simple for humans and very difficult for software.
The use of big data in market research has to be about improving insight and not simply counting the greatest number of online posts possible
It is not possible for human eyes to scan every message for full analysis. But conventional software cannot perform the task either. DataSift bridges this divide with a technique it calls machine learning. This involves human specialists providing a computer program with repeated definitions of a sentiment or entity until the program can apply those categories automatically. Machine learning is essentially training by rote and resembles a high-technology version of teaching a dog to sit.
This raised level of understanding by the software is combined with DataSift’s analysis of data by specific demographics. While the information gushing out of social media remains anonymous, market researchers must know which demographic they are dealing with.
“There is an historic problem with using social media for market research,” Mr Barker explains. “You need to be sure you are looking at a representative sample, not just random opinions.” Market research clients specify which audience they are interested in, so the analysis must represent the opinions of their customer base and not just the general public.
Market research techniques have always tried to isolate segments of the public through focus groups and opinion panels. So the use of big data in market research has to be about improving insight and not simply counting the greatest number of online posts possible.
DataSift’s social media partners span 24 networks and include popular blogging tools such as WordPress. Its latest service, called Vedo, allows DataSift clients to track their target audience right across this online world.
Consumers are trawling the internet for advice long before they arrive on a company website to make a purchase. So DataSift aims to open up that journey for analysis. For example, this may involve observing the consumer visiting sites such as TripAdvisor before they choose a travel destination. “You must be able to look right across the spectrum when you study a consumer,” says Mr Barker, “because today more than half of a consumer journey is done before they contact the supplier.”
He sees his work as taking market research far beyond the usual public perception of a forlorn figure accosting strangers while clutching a clipboard. Social media analysis has opened a whole new window on to the world of customer behaviour. “We are using data that simply did not exist a decade ago, but we do need the skills to turn it into valid survey results.” So DataSift applies classic analytical judgment to the big numbers.
This need to discriminate and focus on the right slice of society when confronting big data is a driver for Gateshead-based Colourtext. This company specialises in what founder Jason Brownlee terms “social listening”.
Colourtext identifies panels up to 5,000 subjects who are active on social media and represent relevant characteristics such as age, gender or geographical location. By selecting a precise demographic, Colourtext can be confident that these social-listening exercises are not just reflecting the general noise of social media.
One of its panels, representing UK millennials, those born between 1980 and the early-2000s, generated 400,000 Twitter posts in a month. Colourtext uses its own software to analyse what is being mentioned in these tweets and also widens this by looking at which hashtags proved popular. The key to it all, says Mr Brownlee, “is choosing who you listen to and not collecting everything out there on a particular topic of interest”.
James Murphy, head of insight at marketing services agency Dissident, agrees that social listening has a big role to play in market research. But he cautions that the use of social listening is embryonic in market research and it should not be treated as single source of truth. “Social media is a volatile tool. You need to get corroborative material, to mix the social listening with old-fashioned market research tools, asking questions in focus groups or running surveys,” he concludes.
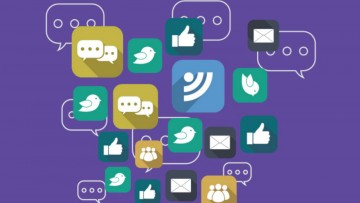