The democratisation of AI: benefits and challenges
It’s an exciting emerging technology – but how can leaders spread it throughout a business safely?
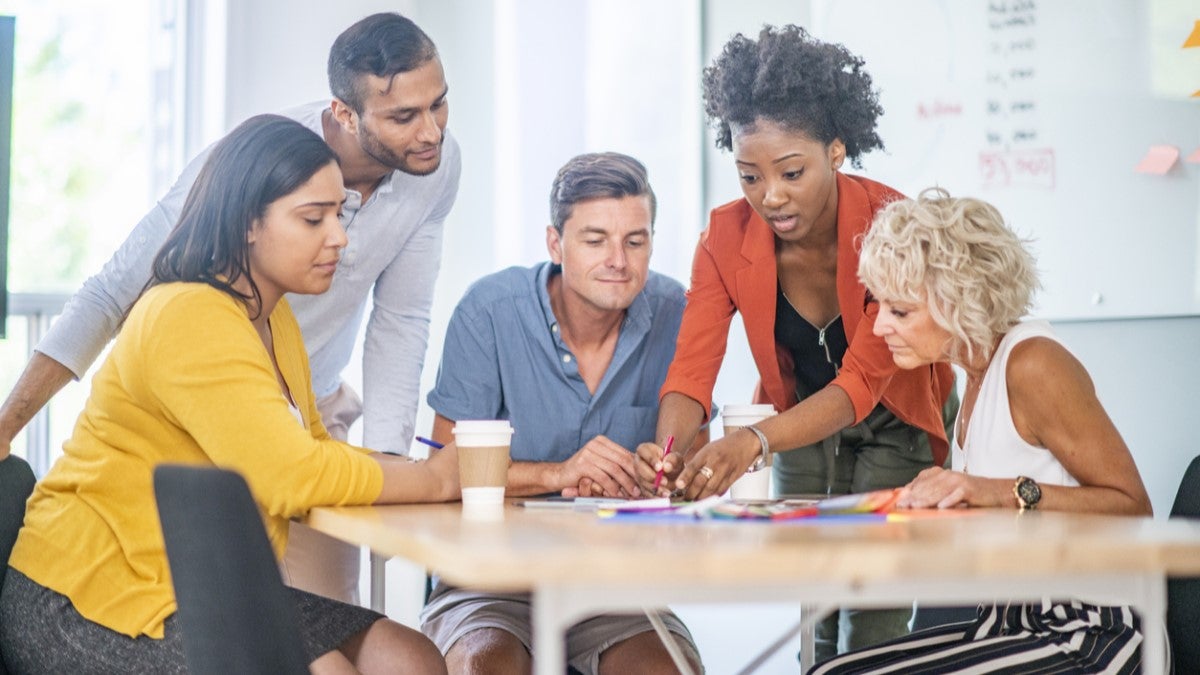
When the starting gun was fired on the generative AI revolution with the November 2022 release of ChatGPT, it set about a domino effect across the business world. Democratising access to AI puts powerful tools in the hands of every business – and every employee within an organisation.
The adoption of such tools is set to account for a 7% jolt to global GDP in the coming decade, according to Goldman Sachs. But it also comes with risks alongside potential rewards.
Navigating through those pitfalls to find the potential of generative AI is a key challenge for businesses operating in the years to come. This is still a new technology, with rules and best-use cases drawn up and rewritten seemingly daily.
Risks and rewards
Whether a company decides to dive into the AI revolution head-first or not, it’s likely already in use within the business. A survey of workers in the US and UK by Asana found that 46% of American workers use AI at least once a week in their roles, while 29% of British workers do the same.
A recent survey by the Chartered Management Institute, the professional body for management and leadership, highlights how executives are as worried about the potential of AI as they are enthralled by it. Three-quarters of managers are concerned about the security and privacy risks of using AI technologies, with less than one in 10 managers confident that their staff are adequately trained to use the tools.
“We are moving into a new phase of AI’s role in our workplaces,” says Saket Srivastava, chief information officer at Asana. “Our study shows that more employees are now embracing AI at work. Employees see the potential of AI to save time and help them focus on more strategic tasks. However, there are clear obstacles, with some employees harbouring concerns about how their AI use could be perceived by peers and managers.”
AI in action
One organisation using generative AI to try and ease the amount of grunt work on employees and improve productivity is Leicester-based marketing agency Go Inspire. The company is utilising large language models (LLMs) to try and more easily identify, then interpret, trends in their clients’ data. “Currently, this is a time-intensive task undertaken by a data analyst, using different techniques such as clustering and correlation, followed by writing everything up,” says Andrew Adkins, director of insight and analytics at Go Inspire.
Go Inspire has seen benefits, as well as pain points, in accessing and rolling out AI throughout the business. “Based on our initial testing of the LLMs, we’re seeing that the only way to get usable results is to be extremely specific with the questions you ask,” says Adkins. “If you give it a few million order lines and customer records and ask it to tell you what significant customer trends exist, the results are usually spurious and don’t pass the ‘so what’ test.”
However, by carefully dictating the terms through which the AI can work, Adkins and Go Inspire have seen some success. “While it doesn’t feel like a creative process, the results we get are better and more extensive than having a person analyse the data,” he says.
Good rules to get results
Go Inspire is one company dipping a toe in the AI waters and seeing where that takes the business. But for Assaf Ronen, chief platform officer at Payoneer, taking a more deliberate approach is the way his business plans to analyse and adopt AI. “The starting point of defining any AI strategy is to consider the value you’re looking to bring, who your customers are and what their needs are in the long term,” he says. “Confirming all those elements will allow you to set out your AI strategy in a way that aligns with your business objectives.”
It’s also important to brief executives on how AI works, as they’re the ones who will be responsible if anything goes wrong. “It’s important to balance the short-term gains in a way that builds trust and keeps data safe,” says Ronen. He notes that eager developers are uploading customer data to public generative AI services like ChatGPT without considering what happens to that data when it’s placed there – an issue that Samsung learnt too late when employees began unilaterally using ChatGPT, unwittingly uploading proprietary information to the chatbot. Since then, Samsung has banned its use within the organisation.
Having those rules written down is important, but lacking. The Asana survey highlights that half of workers want their organisations to provide more guidance on how AI can and can’t be used. “Employees can’t navigate this AI shift alone,” says Srivastava. “They need clear guidelines to understand AI’s role in their functions, along with tailored training and accessible technologies to fully harness AI’s capabilities. Organisations that get this right will leverage AI in a way that unlocks new levels of human ingenuity.”
Should organisations use public generative AI applications?
It’s the potentially multibillion-dollar question: adapt or risk falling behind?
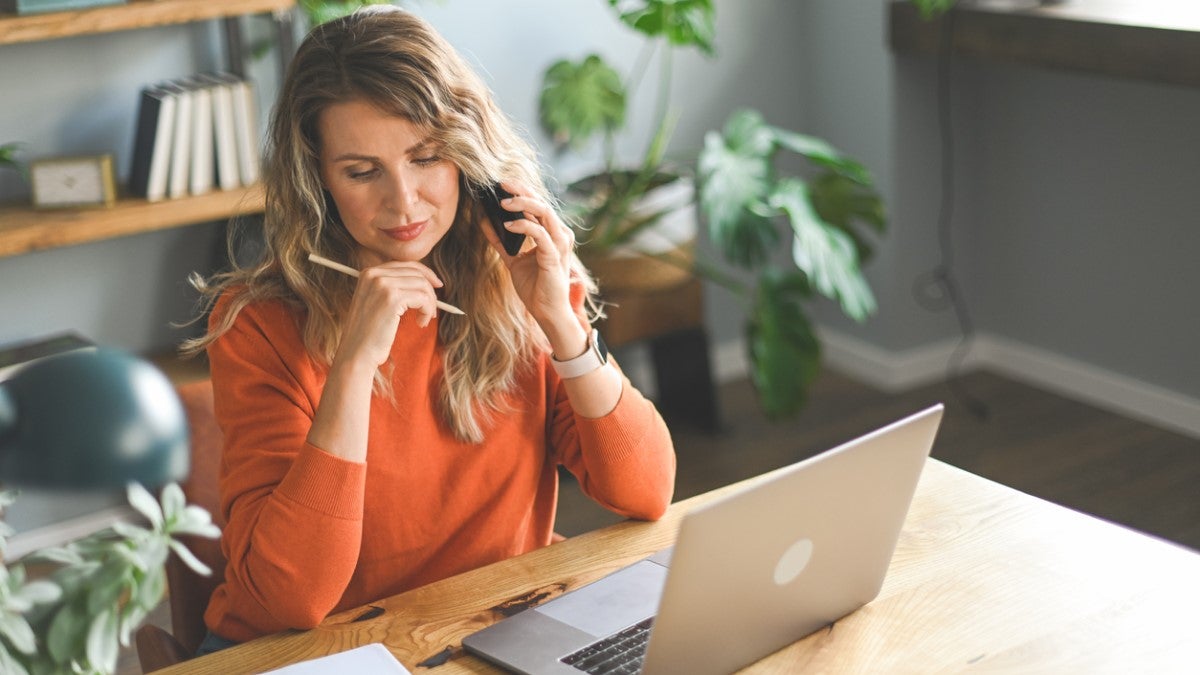
It’s a quandary that is vexing C-suites around the world: should we ignore or join the all-powerful AI revolution?
There’s a risk that if businesses opt out of adopting AI they could get left behind. OpenAI’s own data suggests that 80% of the Fortune 500 are using ChatGPT, benefiting from the productivity gains that generative AI provides. A recent McKinsey report calculates that generative AI could boost productivity by $4.4tn (£3.6tn) every year.
But using public generative AI applications isn’t without risk. “Public large language models like ChatGPT can bring a range of benefits to businesses. But they can also present risks, including to data privacy and security, and raise questions about explainability and bias,” says Arun Ramchandran, who heads up the Generative AI Consulting and Practice Unit at IT services company Hexaware.
As a result, some businesses are looking into a halfway house that allows them to benefit from the power that generative AI provides in unlocking productivity gains, while keeping their data safe. That halfway house is to create a custom-based LLM. “Developing tailored LLMs helps businesses to move away from this – but it’s easier said than done,” admits Ramchandran.
Going private
Developing and training an LLM is a daunting task and Ramchandran says that there are several obstacles on the path to achieve it – “the most obvious of which is the sheer costs that can be involved.” That’s about training the model on computationally heavy (and expensive) graphics processing units, the computer chips used to power AI tools that are in short supply worldwide.
Once the model is up and running, there will be costs to constantly update its training data, while the power, energy and resource demands are significant. Of course, training doesn’t stop with the AI. Your team needs to be taught how to use it properly. “As generative AI is still emerging, most businesses will have a skills gap to address before they can move forward with it,” says Ramchandran. This could cover everything from prompt engineers on the front line to AI leadership figures who can drive strategy and hold overall responsibility. There’s a whole set of skills needed before companies even think about creating their own LLM model.”
One development that may help make the case for using public generative AI tools is the recent release of ChatGPT’s enterprise version. “The security community has raised concerns about the potential for employee misuse to cause data leakage into ChatGPT, because that risks the model training on sensitive information and then using then it for other prompts,” says Jamie Moles, senior technical manager at ExtraHop, and a cybersecurity expert with 30 years’ experience in the industry.
ChatGPT changes the game (again)
The enterprise version of ChatGPT, which includes a higher level of security and baked-in encryption, promises not to use data which has been input to train the model. This has changed the game. It’s likely, then, that many businesses will choose to embrace the publicly available platforms such as ChatGPT that offer convenience over customisability.
But there is a case for going bespoke when considering how to integrate an AI system into your business, particularly in sectors where the integrity of your data handling is vital – such as in scientific research and development, or medicine. Beyond insulating yourself against the risk of data leakage, proprietary models have a further benefit: they’re trained on a narrower set of data, which reduces the risks of the model misfiring because of bad training data. In many instances, the hurdles thrown up by developing your own model can be outweighed by the benefits of having control over your data, making it a sensible, obvious choice.
Going it alone entirely can be tricky, which is why Ramchandran recommends being intelligent about buying in support to fill the gaps in expertise that will likely arise because very few people have capably built such generative AI tools before. That external expertise is also vital to check whether your model works correctly: validating its outputs before putting it into production is important for your bottom line. “Organisations can of course look to develop their own generative AI models, but they also need to be realistic that they will likely need to call on third-party expertise at some point,” he says. “Doing this will put them on the path to overcome the potential challenges and reap the rewards.”
How have AI priorities changed?
Before the generative AI conversation took off, few organisations had adopted AI as a critical part of any business function
Foundations for innovation: unlocking the power of generative AI
Data-forward businesses are looking at the potential of AI to innovate – but how can they build the right data foundations?
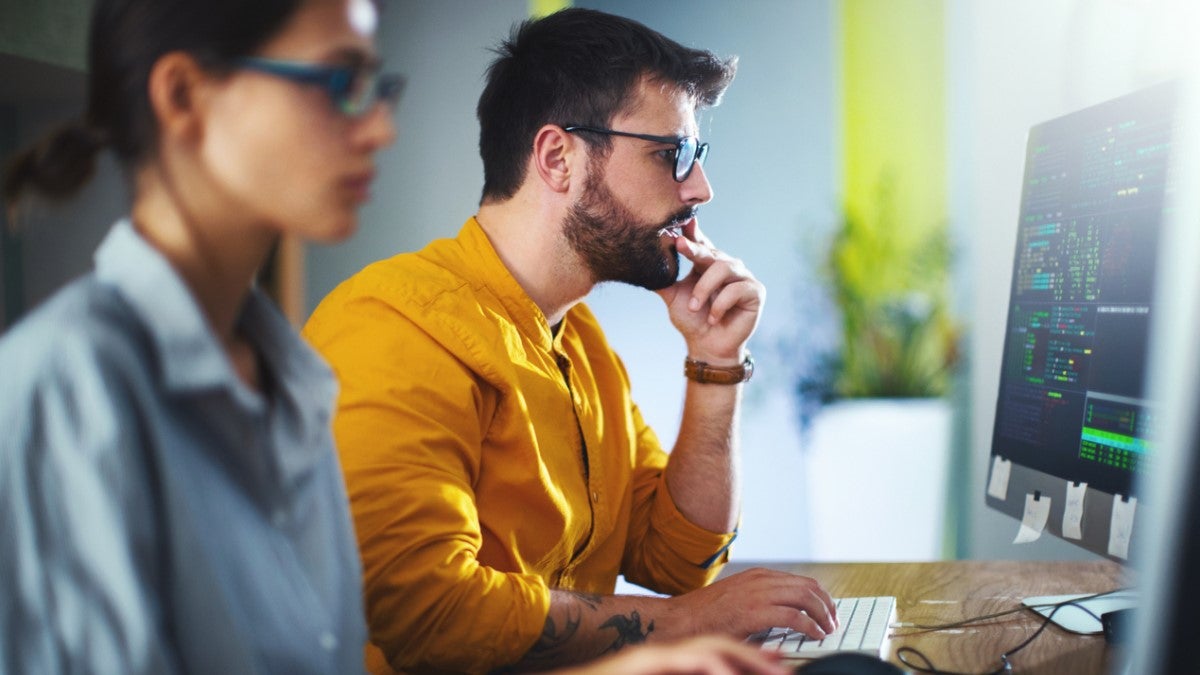
The race to roll out AI has plenty of competitors. But when many are rushing to adopt the new, revolutionary technology, the real winners will be those who take care to build the right data foundations to integrate AI safely and meaningfully.
Doing that is easier said than done. Dael Williamson, chief technology officer at Databricks in Europe, the Middle East and Africa, answers key questions about how to responsibly innovate using AI – and how to build a data infrastructure that will lead to long-term success.
Where are companies at present in terms of using generative AI?
The short answer is there’s a spectrum of use cases. Most companies have done something – some form of use case or proof of concept with OpenAI or a similar tool. But with the fast-changing world of AI and the development of large language models (LLMs), many would be forgiven for wanting to pause for thought on which tool is the right one for them.
At Databricks, we have open-source roots and our strategy is to not have just one model. The reason for that is two-fold: one, protecting intellectual property, and two, how can one model be very specific to a single area?
There’s a concept called ‘data contamination’. Essentially, the bigger the model, the more likely the datasets are contaminated. If a company creates a very specific model with a very specific dataset, it can offset a lot of that contamination.
We’re starting to see companies say: ‘We need to create a culture of experimentation and education.’ There is a duality happening at the moment where customers want to experiment with LLMs and generative AI but also want to ensure that their plans and budgets for this year are locked in. What this means is they are willing to take ‘educated experiments’ by trialling various LLMs and AI tools, but want to do so with governance built in from the beginning.
How can those leaders who are experimenting in the hope of implementing generative AI more widely build the correct data foundations?
The first consideration is where all of the company data resides. At Databricks, we believe that everything starts with data. I ask customers: ‘What is your data gravity? Where is it all sitting?’
I’ll ask them to pretend they’re a retail store and data is their product. They should imagine they’re putting these things on the shelf, and people are coming to shop at the store. Are they a niche retailer or a wholesaler?
Many companies will start to say they’re 75% along the way in one direction, and then I’ll say: ‘I want you to think about video, documents, images, code and Excel.’ Suddenly that number drops to 10%. Leaders need to start thinking about the total addressable data in the organisation.
Then leaders need to think about how to consolidate it. And that doesn’t mean centralising all the data. It just means putting it in a standard, accessible format. Organisations also need to have some degree of governance over their data. They need to know how it’s moving, what it’s doing and where it’s flowing.
How important will governance be? And how should business leaders put it in place?
Governance is going to be very important. Organisations will also need to know what the existing models are and if they can govern them. CIOs and business leaders need to ask themselves: ‘Do we know the training data that built the model? Do we know the inputs? Do we know the outputs?’ That is a difficult question to answer with the current models.
I think the majority of investment this year will focus on education and experimenting with building small models that companies can get better at testing and explaining. That solves intellectual property considerations. But there are also issues to address around end-to-end testing and responsible AI. How do we measure bias?
How does Databricks help organisations build the right foundations?
What’s great about Databricks is we’re a data intelligence platform. With DatabricksIQ, for example, organisations can capture business context and semantics, connecting to underlying data. This means companies can monitor all kinds of data through Databricks and govern their models effectively. What that means is companies can start to build generative AI applications. There’s a big upside there.
We’re starting to see companies experiment by using LLMs to interface with their data. We have customers who have built incredible new data intelligence platforms and business dashboards.
That helps with a common problem. We’ve seen that many companies are striving to build a data intelligence platform. It doesn’t matter what industry they’re in: everyone is doing the same thing. But the part they often miss is ‘to do what?’. We can help them take that step back and see the insights that answer that foundational question.
How data governance frameworks will need to evolve for AI
Innovating while maintaining the organisation’s reputation and legal standing is vital
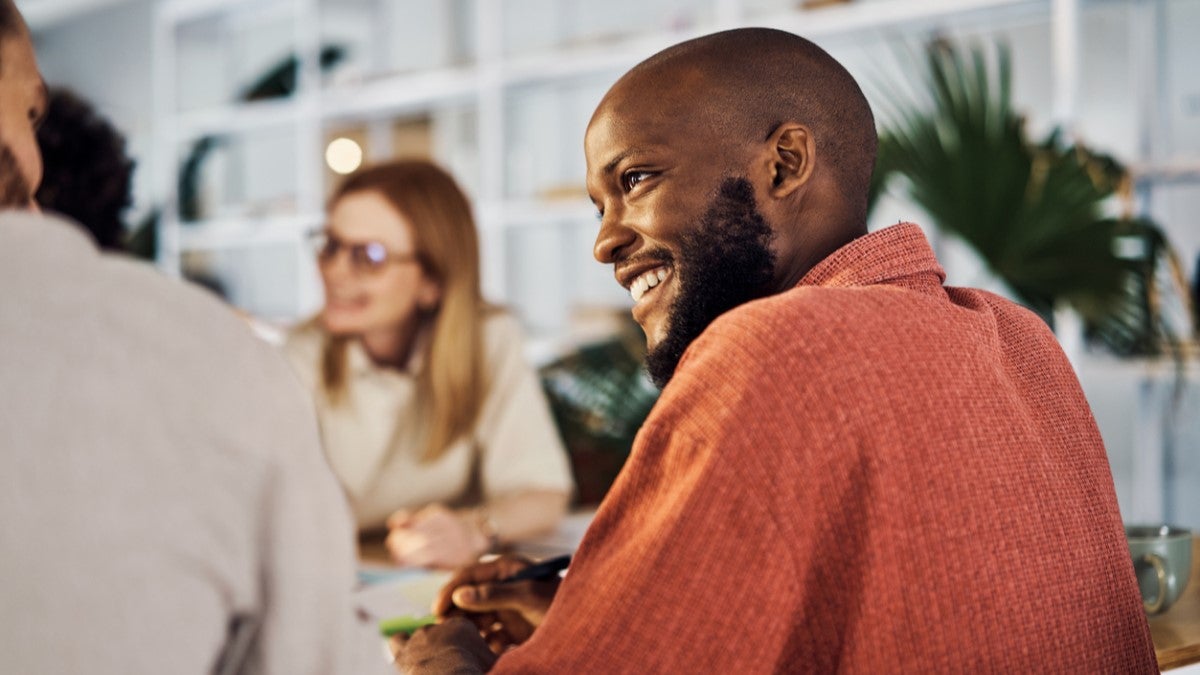
The 2018 introduction of the general data protection regulation (GDPR) was a momentous event for businesses – and not always for the best reasons. It created a mountain of paperwork and requirements to meet the regulations, or risk falling foul of the rules and being hit with massive fines.
The current AI revolution is equally significant and the opportunities provided by integrating AI into workflows could be transformative. But AI is a double-edged sword, with real risks to organisations that don’t establish an updated and consistent data governance framework in place to ensure guardrails are placed around the technology.
“There’s a fine line between delivering innovative AI experiences for employees and customers, and ensuring a high level of security and privacy,” says Sophie Stalla-Bourdillon, senior privacy counsel and legal engineer at data security platform Immuta. “This is especially true for generative AI, which exemplifies how innovation is rapidly disrupting practices, posing enormous risks to data security and privacy.”
Stick or twist?
With such a transformative technology at such a young stage of its development, bumps in the road have already appeared. Take the training data that powers the ‘brains’ of AI tools that create text, images or video. Questions remain about where the data for them was sourced, and whether the companies developing the models obtained the proper rights to use them. Multiple court cases are ongoing about the issue, with artists and copyright-holders, including Hollywood comedian Sarah Silverman, claiming their rights have been infringed.
For that reason, many companies are shying away from using the outputs of generative AI – at least publicly. It’s part of a broader caution around the technology, recognising its transformative effect while also being aware that the rules about its use are still being written.
That’s before even considering what happens if organisations feed models proprietary information that is then used to train AI tools that are used by competitors – although a new raft of enterprise-specific tools should help avoid that.
Stalla-Bourdillon points out that many companies want further official guidance before they leap into the world of AI – or at least more surety. No business wants to go to the expense of refiguring the way their company works to account for the new AI revolution, only to find that it must then retool its entire operations to ensure it’s compliant with a new raft of regulations.
The legal case
Many countries around the world are planning to introduce legislation dictating how and where AI can be used, and the data that trains it – and comes out of the models – can be deployed. Leading the way, and most geographically relevant to many British businesses because of its proximity and prior precedent of acting as a de facto global regulator, is the European Union.
The EU’s AI Act is making its way through the legislative process and is likely to take force in the coming years. “The EU AI Act is an important opportunity to provide much-needed direction, although it will take time to implement,” says Stalla-Bourdillon.
While waiting for the national-level rules to be drawn up, organisations should work quickly to ensure they have internal standards that are met sufficiently. “Many of the risks posed by AI tools are probably already covered in existing information security policies – such as not exposing proprietary data to unmanaged third-party sites or parties – but the excitement around the technology has caused these guidelines to be temporarily forgotten,” says Matt Hammond, software architect and founder of tech firm Talk Think Do.
But there aren’t solely risks. There are data governance opportunities in AI, too. Security and risk management was the top tangible benefit of AI executives pinpointed in a 2022 Databricks survey, while executives were energised by the future opportunity of using it to detect fraud and improve cybersecurity.
Building up resilience
Hammond foresees a future when AI policies will be considered as essential as data protection policies have become since the advent of the GDPR. And so it makes sense, according to him and to Caroline Carruthers, CEO of data consultancy Carruthers and Jackson, to start thinking about it now, rather than later.
“If tech leaders want to best navigate the data governance challenges associated with AI, they have to see it for what it is: another tool in the arsenal that uses data to create better business outcomes,” says Carruthers. “In practice, that means any organisation looking to use AI needs to get the basics of data governance right, creating a governance framework which is easy to understand and constantly updated to take into account the latest regulations.”
Carruthers suggests going slowly and piecemeal, rather than trying to shoehorn all the potential issues that could arise into a data governance framework. “There needs to be a recognition that we simply don’t know how far AI technology will go within business settings,” she says. “Any transformation should be implemented in bite-sized steps, with the ability to roll back a step if the consequences are not as expected.”