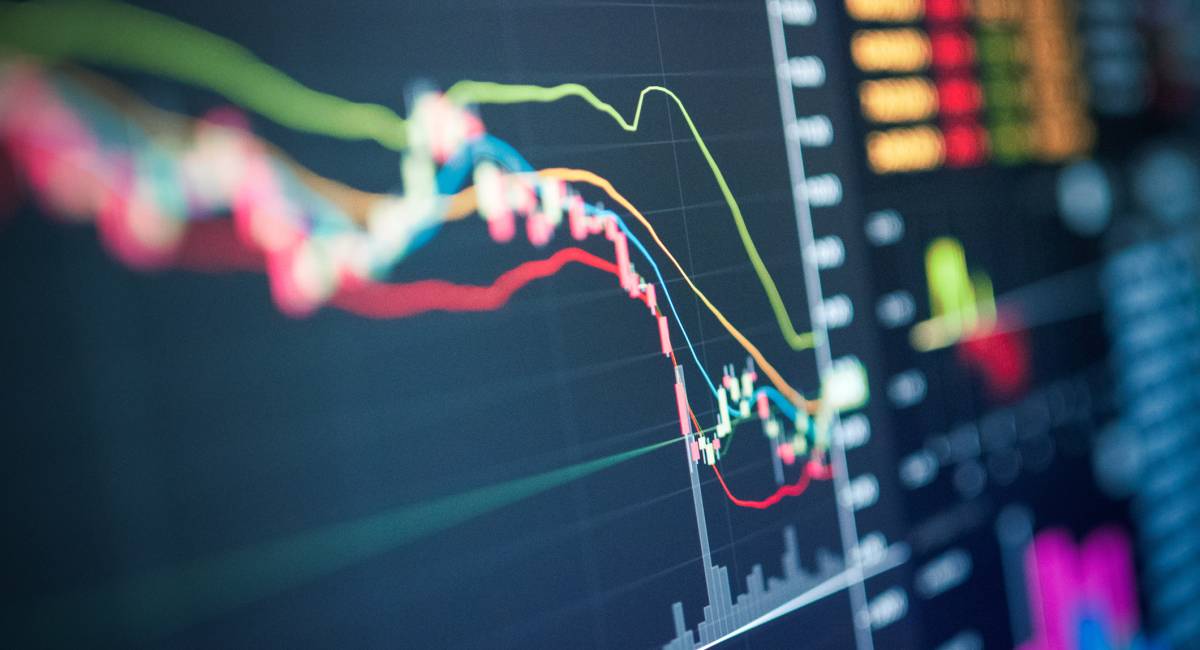
In the leafy enclaves of Boston, Mike Chen and his team have taught machines to read Mandarin slang. They know Chinese investors often use homonyms, pairs of words with the same spelling but different meanings, to trick state sensors on public forums. By cracking this code, the machines can find out what investors really think about China’s leading companies.
This is just one of several linguistics projects that Chen, director of sustainable investing at PanAgora asset management, has developed using machine-learning algorithms, which can identify patterns from the data they receive and learn from their own results. When a company releases an update, these algorithms enable PanAgora to analyse the reaction from its stakeholders and the public.
Similar advances are being made across the investment community. Investors are using machine learning to mine all kinds of information, from the minutiae of earnings disclosures to the content of LinkedIn posts. Using this so-called sentimental data, they can sift through the hype around environmental, social and governance (ESG) issues and get an accurate picture of a company’s credentials.
“By understanding what people are saying about these companies, we can get a true picture of their perceived brand value,” says Chen. “Not only that, but we may be able to detect whether managers are ‘greenwashing’ when they talk about their firm’s ESG policy.”
Across the investment community, researchers and engineers are using machine learning in equally disruptive wys. They’re analysing linguistic information from content, including earnings disclosures to LinkedIn posts, using sentimental data to see whether a company is truly committed to ESG and what sort of impact this commitment has on its stakeholders.
The value of this intelligence is huge. Around a third of all assets under professional management are now subject to ESG criteria. In the second quarter of 2020, when the world was reeling from the coronavirus crisis, investors poured more than $70 billion into green stocks. As issues such as climate change and social justice gain traction, and US President Joe Biden’s progressive regime replaces the ESG-sceptic Trump administration, this popularity looks set to grow still further.
To critics, however, the methods used to evaluate ESG credentials have not kept pace with demand. Until now the ESG ratings sector has been dominated by a handful of providers, such as MSCI, FTSE Russell and Sustainalytics. Each provider has its own rigorous set of metrics, but with no universal standard for what constitutes “good ESG”, their methodology, and thus their ratings, differ markedly.
Some providers score companies on an absolute basis, so everyone is judged by the same criteria, but others score relatively, which can reward the least bad companies in less progressive industries. The flaws in this approach were highlighted last summer when fast-fashion retailer Boohoo received an AA rating from MSCI just weeks before reports that some of its workers were allegedly being paid less than the minimum wage.
The data these providers rely on is also heavily influenced by periodic corporate disclosures. This data isn’t just prone to bias, as companies omit the factors that paint them in a bad light, it is also backward looking. If a company hired a new female board member 11 months ago, how can anyone know whether this affects the market now?
Making ESG data more accessible
As these weaknesses have become more obvious, machine learning has become more democratic. The advent of cheaper off-the-shelf algorithms, combined with advances in computational power, mean investment funds and the analysts who serve independent financial advisers can create their own machine-learning models to process huge amounts of data, both financial and non-financial, in real time.
Key to these models is natural language processing (NLP), a subset of machine learning that enables machines to understand human linguistic patterns. Using NLP, researchers can go beyond traditional market reports to analyse both written and verbal communication to understand how ESG commitments are both presented and received.
Analysts mostly use NLP to analyse the language companies themselves use; whether their declarations are concrete or vague, whether they use the first person or take refuge in the third.
By using machine learning applied to the news, an investment manager can effectively highlight the exact ESG actions a company is taking to promote positive impact
The team at HSBC Global Research in London, for example, uses linguistic analysis to sift through corporate earnings calls. Mark McDonald, head of data science and analytics, says: “One of our main focus areas is how the presenters handle impromptu questions from analysts, as the answers are often much less positive than the pre-prepared statement at the start. This can give a truer picture and you can aggregate the sentiment across markets and regions.”
Other firms are focusing on how external parties react to these statements. They comb thousands of news stories to get instant reaction and often combine this with comment scraped from social media.
At Act Analytics in Toronto, researchers have trained a machine-learning algorithm to scour a carefully curated list of sources from News API, a compendium of around 30,000 real-time outlets.
“By its nature, real-time news is meant to provoke some sort of response, whether positive or negative, since that’s what sells,” says Act Analytics’ head of ESG ratings Elgin Chau. “What needs to be determined is the degree to which an event is material and the extent of its impact on asset prices. By applying our algorithm across multiple news sources, we can calculate an aggregate sentiment score for a particular event for a particular company.
“Much of ESG portfolio performance relies on conjecture or anecdotes. Sometimes you get some ridiculous reports that say your portfolio has taken 200 cars off the road or planted 1,000 trees. These are essentially soundbites, which can’t really be measured accurately and rely on a ratings provider’s subjective interpretation of a company’s corporate disclosures. In other words, these types of reports are imperfect proxies for a portfolio’s actual ESG performance.
“By using machine learning applied to the news, an investment manager can effectively highlight the exact ESG actions a company is taking to promote positive impact.”
How machine learning is complementing data
Data providers need not be worried by this trend. Machine-learning advocates agree that it will not replace traditional data sources, rather the algorithms will work to analyse these sources more effectively and put the data into context. As Chen points out, investors can now gauge not just the numbers that a company puts out, but the “believability” of its sustainability planning.
As consumers and stock-pickers pour evermore money into ethical companies, so the potential for greenwashing will increase. Just this month, research by the UK’s Competition and Markets Authority found that four in ten corporate websites were offering misleading environmental information on their websites.
With machine learning, investors can now find the truth behind these claims. Quite literally, they can read between the lines.
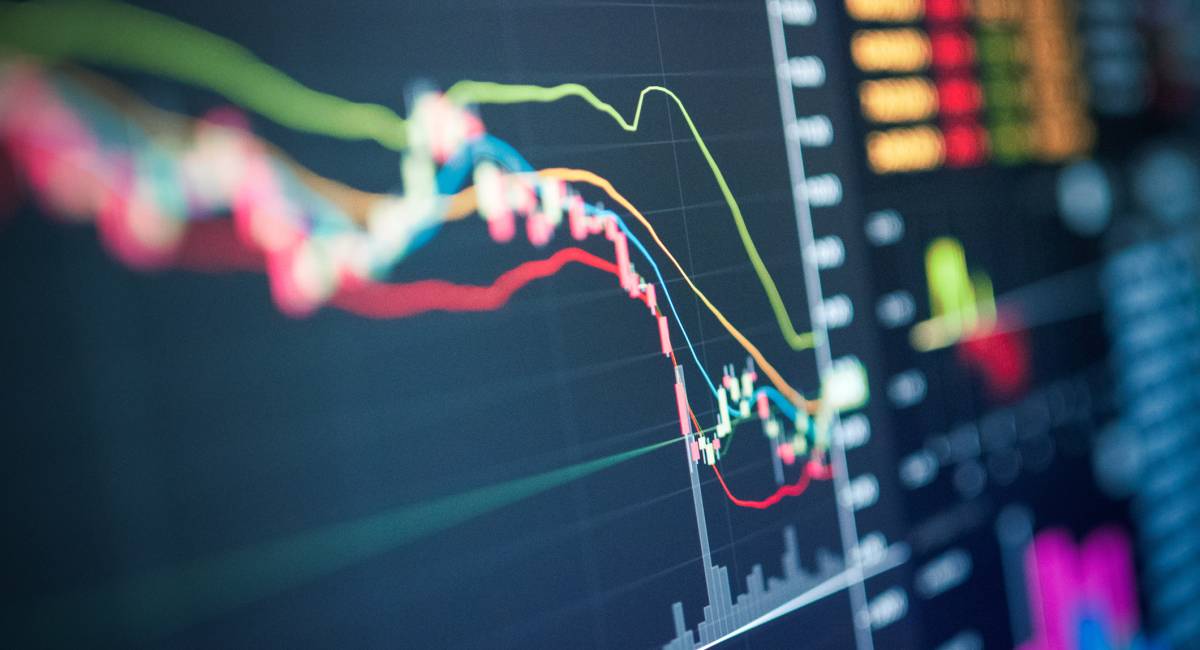
In the leafy enclaves of Boston, Mike Chen and his team have taught machines to read Mandarin slang. They know Chinese investors often use homonyms, pairs of words with the same spelling but different meanings, to trick state sensors on public forums. By cracking this code, the machines can find out what investors really think about China’s leading companies.
This is just one of several linguistics projects that Chen, director of sustainable investing at PanAgora asset management, has developed using machine-learning algorithms, which can identify patterns from the data they receive and learn from their own results. When a company releases an update, these algorithms enable PanAgora to analyse the reaction from its stakeholders and the public.
Similar advances are being made across the investment community. Investors are using machine learning to mine all kinds of information, from the minutiae of earnings disclosures to the content of LinkedIn posts. Using this so-called sentimental data, they can sift through the hype around environmental, social and governance (ESG) issues and get an accurate picture of a company’s credentials.